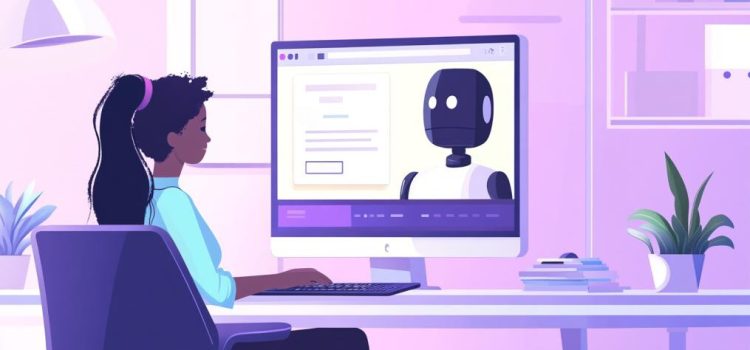
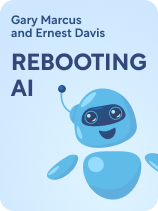
This article is an excerpt from the Shortform book guide to "Rebooting AI" by Gary Marcus and Ernest Davis. Shortform has the world's best summaries and analyses of books you should be reading.
Like this article? Sign up for a free trial here.
What is narrow AI? How does it operate? How does it differ from true intelligence?
In their book Rebooting AI, Gary Marcus and Ernest Davis explore the state of artificial intelligence. They explain how narrow AI works, its benefits, and its shortcomings. The authors explain the mechanics of neural networks and machine learning, highlighting their widespread applications and limitations.
Keep reading to uncover the fascinating details behind today’s technology and its potential future.
Narrow AI
In their book, Davis and Marcus share their assessment of narrow AI. But, what is narrow AI, and how does it work? The current paradigm of AI development combines artificial “neural networks” that can be trained to produce desired outputs when they’re fed vast amounts of data. The authors describe how this process developed, the way that it permeates—and benefits—modern technology, and what it lacks in terms of reaching true intelligence.
(Shortform note: A neural network is a computer model composed of computational “nodes” that simulate the behavior of neurons in the brain. In contrast to a computer’s traditional use of binary signals, each node in a neural network fires according to a combination of inputs whose relative proportion and influence are heightened or lessened as the system “learns” to produce desired outputs. Despite the terminology used by software engineers, neural networks don’t truly embody the full range of behaviors of neurons in the brain, which leads Davis and Marcus to suggest that the term “neural network” itself is misleading.)
In the 20th century, software developers had to manually input the instructions for anything a computer had to do. When it came to simulating intelligence, this process was unfeasible, so researchers developed the first neural networks that could perform “intelligent” tasks by analyzing large amounts of data. This was also a cumbersome approach until the early 2010s, when computer processors grew fast enough to cope with the huge datasets that became available. Neural networks became deeper and deeper—meaning more computational layers could be added between inputs such as queries and the computer’s output.
(Shortform note: Contemporary rapid progress on AI has taken many outside observers by surprise. In The Future Is Faster Than You Think, Peter H. Diamandis and Steven Kotler explain that the explosion of new developments we’re seeing is due not only to the improvement of technology, but to how different technological improvements are merging to further accelerate progress and innovation. Whereas Davis and Marcus cite the combination of more powerful processors with ever-growing amounts of data, modern AI-driven companies such as Google are working to merge competing AI platforms to produce even faster, more powerful results.)
Power in Statistics
Marcus and Davis explain that, despite its complexity, machine learning relies entirely on statistical probabilities to determine its outputs. Nevertheless, it’s now found everywhere, mostly to positive results, from the system that recommends movies on your streaming service to the facial recognition software used in airport security. Modern search engines wouldn’t work at all without big data and machine learning, nor would many of the programs we now use, which can be “taught” to computers much quicker than they could ever be keyed in by hand. Thanks to these successes, neural networks and machine learning are the driving force behind AI development as the industry presently stands.
(Shortform note: Marcus and Davis discuss machine learning and big data as two separate pieces of the AI puzzle, but in practice, neither one works without the other. Their results speak for themselves, as big-data-driven systems have proved useful in financial risk and fraud prevention, helping doctors make better medical diagnoses, and identifying communities in need of humanitarian aid. Widespread use of big data has raised public concerns about privacy and security, which have been somewhat addressed by government regulation and increased data usage transparency on the part of some corporations.)
Nevertheless, Davis and Marcus insist that machine learning has one major flaw—statistical correlation doesn’t lead to true intelligence; it can only create the illusion of understanding. Some developers have tried to overcome this hurdle by leveraging the human understanding of their users. For instance, the software behind social media sites learns to prioritize links and posts that human users click on more often. When that strategy leads to poor results, such as perpetuating bias or misleading information, engineers try to solve it problem-by-problem without addressing the underlying issue—that the AI running the social media site has no real comprehension of what the information it’s spreading really means.
(Shortform note: Though not foreseen by Davis and Marcus, the most public way that AI developers have leveraged human intelligence to enhance AI is by using human-created text, music, and visual art to train systems such as ChatGPT, DALL-E, and MuseNet to generate human-level AI content. This has caused an uproar among creative professionals, many of whom view the use of their work to train AI output as a form of plagiarism. The New York Times filed a lawsuit against OpenAI for its use of this practice, while Universal Music Group removed its content from TikTok in a bid to defend its artists from AI copyright violations.)
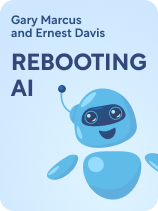
———End of Preview———
Like what you just read? Read the rest of the world's best book summary and analysis of Gary Marcus and Ernest Davis's "Rebooting AI" at Shortform.
Here's what you'll find in our full Rebooting AI summary:
- That AI proponents oversell what modern AI can accomplish
- How AI in its current form underdelivers on its creators’ promises
- The real danger—and promise—of artificial intelligence