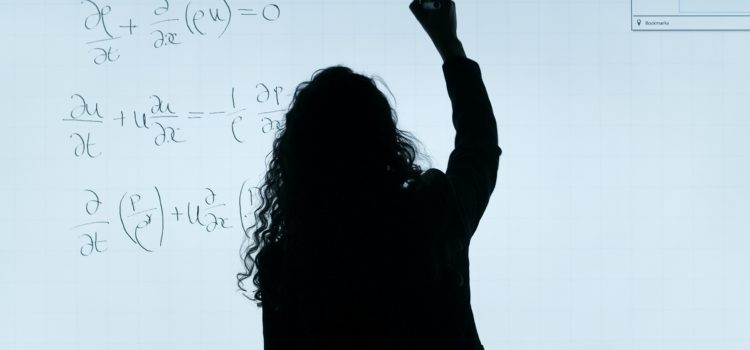
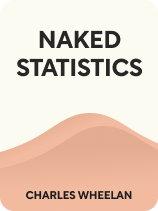
This article is an excerpt from the Shortform book guide to "Naked Statistics" by Charles Wheelan. Shortform has the world's best summaries and analyses of books you should be reading.
Like this article? Sign up for a free trial here .
What are Type I and Type II errors in hypothesis testing? How can you minimize your chances of accepting a wrong hypothesis?
Type I and Type II errors both relate to the result of the null hypothesis. A Type I error occurs when the null hypothesis is mistakenly rejected, whereas a Type II error occurs when the null hypothesis is mistakenly accepted.
Keep reading to learn about the difference between a Type I and a Type II error, and how to reduce your chances of making both.
Type l and Type ll Errors—Explained
Type I errors are false positives, which means we reject a null hypothesis that is actually true (and accept an alternative hypothesis that is actually false). For example, if the absorption of our vitamin company’s new formula wasn’t any better than the previous formula, but their study led them to conclude that it was, they would be making a Type l error.
Type lI errors are false negatives, which means we accept a null hypothesis that is actually false (and reject an alternative hypothesis that is actually true). For example, if the absorption of our vitamin company’s new formula was better than the previous formula, but their study led them to conclude that it wasn’t, they would be making a Type ll error.
Setting your burden of proof (confidence level) high, say, .001, makes it statistically more difficult to reject the null hypothesis because, at that level, you have to be 99.9% certain the alternative hypothesis is true before you can reject the null. This makes you more likely to make a Type ll error by accepting the null hypothesis as true when it’s not.
In contrast, setting your confidence level lower, say, .1, reduces the burden of proof necessary to reject the null hypothesis because you only need to be 90% certain the alternative hypothesis is true before you can reject the null. This makes you more likely to make a Type l error by rejecting a null hypothesis that is actually true (and accepting an alternative hypothesis that is actually false).
Reasons for Type I and Type II errors in Hypothesis Testing A small sample size can be the cause of some Type ll errors. For example, say you were studying whether malaria is more common in mosquitoes in one region than another, but you only collect 20 mosquitoes from each location. It’s possible that you’d miss a statistically significant difference between the larger mosquito populations because you simply didn’t survey enough mosquitoes. Therefore, large sample sizes can help reduce Type ll errors. A significant relationship other than the one you’re studying can also be the cause of some Type l errors. For example, say you’re studying the effects of a healthy lunch initiative on school children’s attention levels in class. Your statistics show a significant relationship between increased attention levels and participation in the healthy lunch program. However, this increased attention may actually be caused (at least in part) by the new outdoor exercise program the school implemented at the same time, and students are focusing better in class after coming in from an outdoor walk. Therefore, careful consideration of possible confounding factors can help reduce some Type l errors. |
Since you can never fully eliminate the possibility of making Type l and Type ll errors, the circumstances around your research and statistical analysis will determine which one you are more willing to accept.
For example, as a medical researcher working on a new vaccine, you might have a very low tolerance for rejecting a true null hypothesis (Type l error). Since people’s health is at stake, you want to be very sure that your vaccine works. Therefore, you might opt for a high confidence level, say, .001, meaning that you are 99.9% confident when you reject the null hypothesis that your vaccine is not effective against a certain malady. In this case, by opting for such a high level of confidence, you increase your chances of making a Type ll error and concluding that your vaccine is not effective when, in fact, it is.
In contrast, you might be more inclined to accept a Type l error as a social sciences researcher looking to implement a recreational therapy program at a local senior center. You might even set your confidence level at .25, meaning that you would be 75% confident in rejecting the null hypothesis that your recreation program does not produce clinically significant results. In this case, your rationale might be that even if the results of the program are not clinically measurable, the community as a whole will still enjoy it, and there is little risk of doing harm.
Remembering Type l and Type ll Errors The concept of Type l and Type ll errors can be tricky to grasp and retain. An article from the National Library of Medicine suggests using the story of the Boy Who Cried Wolf as a memory tool. The first time the boy cries “wolf” in the story, the citizens all come running. This is a Type l error because there is no wolf even though they think there is. We can remember that it’s a Type l error because it happens first in the story. Later, there really is a wolf when the boy cries “wolf,” but no one comes since they don’t believe him. This is a Type ll error, which we can remember because it happens second in the story. |
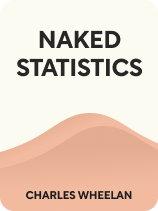
———End of Preview———
Like what you just read? Read the rest of the world's best book summary and analysis of Charles Wheelan's "Naked Statistics" at Shortform .
Here's what you'll find in our full Naked Statistics summary :
- An explanation and breakdown of statistics into digestible terms
- How statistics can inform collective decision-making
- Why learning statistics is an exercise in self-empowerment