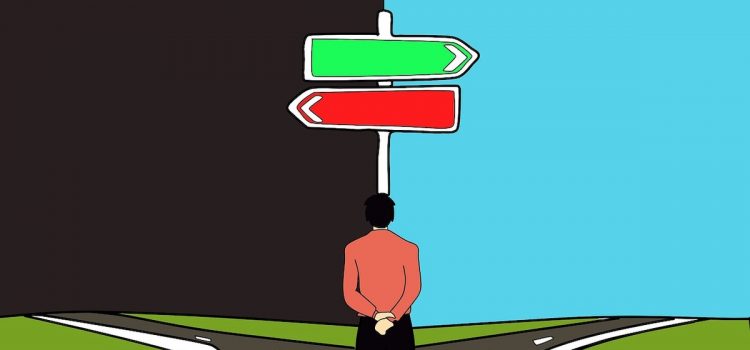
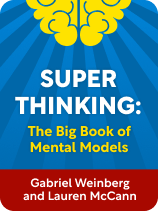
This article is an excerpt from the Shortform book guide to "Super Thinking: The Big Book of Mental Models" by Gabriel Weinberg and Lauren McCann. Shortform has the world's best summaries and analyses of books you should be reading.
Like this article? Sign up for a free trial here.
Why is it so hard to make decisions? What are some ways you can narrow down your options to make it easier to arrive at an optimal choice?
Decision-making is difficult because nobody has access to perfectly accurate, comprehensive sources of information. Rather, our information is often messy and flawed, so we can’t always predict how our decisions will pan out.
In this article, we’ll explore three types of decision-making models to recommend the best choice based on the complexity of the decision.
1. Least Complexity: Pro-Con List
First, let’s look at the best-known type of decision-making models: the pro-con list, which sorts the pros and cons of a decision in parallel columns, making it easier to see the positive and negative consequences. For instance, imagine you’re a lawyer deciding whether to accept a higher-paying position that would require moving away from your family. In this situation, you could draft the following pro-con list of accepting the position:
Popularity notwithstanding, Weinberg and McCann argue that the pro-con list has several flaws:
1. It creates a false dichotomy, since many consequences don’t fit neatly as pros or cons.
2. It fails to weight different pros and cons, so you focus solely on the quantity of pros and cons.
3. The grass-is-greener mentality leads us to naturally overemphasize pros. (Shortform note: While the grass-is-greener mentality inclines us toward decisions that require change, status quo bias—the preference for our current situation over alternatives—inclines us against making decisions which involve substantial change. In such cases, we may be naturally predisposed to overemphasize the cons of change, rather than pros.)
So, while a pro-con list may work well enough for simpler decisions, Weinberg and McCann caution against using it in more complex decisions.
2. More Complexity: Cost-Benefit Analysis
To improve, Weinberg and McCann recommend creating a cost-benefit analysis of your decision. Unlike pro-con lists, a cost-benefit analysis lists the cumulative costs that a decision will incur alongside its cumulative benefits. It thus quantifies the consequences of your decision.
At a rudimentary level, a cost-benefit analysis might weigh individual pros and cons on a scale of 10 to -10, summing these values to recommend a decision—if the total is positive, the decision is worthwhile, and if it’s negative, the cost is too high. Returning to our pro-con list above, you might retool it into the following cost-benefit analysis:
Unlike the pro-con list, the cost-benefit analysis yields a more conclusive verdict: You shouldn’t take the new position, since the cons outweigh the pros.
To further improve your analysis, Weinberg and McCann recommend assigning each cost and benefit an explicit monetary value. For instance, relocating to a new city might cost $10,000, whereas an extra week of paid leave might be worth $3,000.
3. Most Complexity: Decision Trees
Both the pro-con list and the cost-benefit analysis assume that you’ll know what will result from your decision. But Weinberg and McCann recognize that sometimes, there are many possible outcomes, and you have only a rough idea how likely any given outcome is. For instance, when governments institute new policies, they rarely know the exact consequences beforehand.
In such cases, use a decision tree to handle this uncertainty. In addition to assigning monetary values, decision trees also assign probabilities to each outcome, representing the likelihood that it will occur.
To see this in action, let’s continue the lawyer example with a few caveats: First, if you accept the new position, there’s an 80% chance that you’ll thrive and eventually become a partner. Second, the increased pressure might make you crack, so there’s a 20% chance that you’ll end up getting fired at the new job. Finally, you’re 100% certain that staying at your current job will lock you into your current position—an associate—for the foreseeable future.
Here, there are three possibilities: You accept the new job and become a partner, you accept the new job and crack under pressure, or you stay at your current job and remain an associate. Assuming you’ve assigned these three outcomes values of $100,000, -$50,000, and $75,000, respectively, you can create the following decision tree:
This decision tree lists possible decisions in the first node (rectangles), the likelihood of a given outcome in the second node (ovals), and the monetary value of that outcome in the third node (diamonds).
Crucially, decision trees allow us to calculate each decision’s expected value, the average monetary value resulting from a decision. To do so, multiply the likelihood of each outcome by its respective monetary value, and then sum them up for each decision. If your decision has two possible outcomes, your calculation would look like this:
(Outcome 1 likelihood)x(Outcome 1 monetary value) + (Outcome 2 likelihood)x(Outcome 2 monetary value) = (expected value)
For example, you’d calculate the expected value of accepting the new job with this formula:
(0.8)x($100,000) + (0.2)x(-$50,000) = $70,000
By contrast, the expected value of keeping your current job is more straightforward:
(1)x($75,000) = $75,000
Because the expected value of keeping your current job is higher than that of accepting the new job, the decision tree recommends keeping your current job.
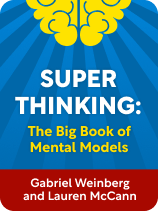
———End of Preview———
Like what you just read? Read the rest of the world's best book summary and analysis of Gabriel Weinberg and Lauren McCann's "Super Thinking: The Big Book of Mental Models" at Shortform.
Here's what you'll find in our full Super Thinking: The Big Book of Mental Models summary:
- How to elevate your everyday reasoning and decision-making skills
- Why you shouldn't rely on intuition when making decisions
- How to deter you opposition from engaging in conflict