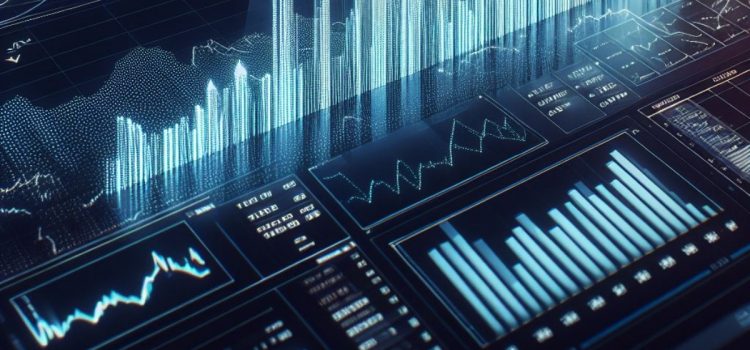
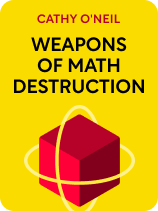
This article is an excerpt from the Shortform book guide to "Weapons of Math Destruction" by Cathy O'Neil. Shortform has the world's best summaries and analyses of books you should be reading.
Like this article? Sign up for a free trial here.
How powerful is math? What’s the difference between benign math models and dangerous math models?
In Weapons of Math Destruction, Cathy O’Neil notes that not all mathematical models are dangerous. When implemented responsibly, mathematical models can be powerful tools that enable organizations to make effective decisions that wouldn’t otherwise be possible.
Below we’ll define mathematical models to show the power of math.
What Are Mathematical Models?
To understand the power of math, you need to know how people and organizations use it. According to O’Neil, a mathematical model is a mathematical simulation of a real-world event. Organizations use mathematical models to make predictions and decisions more efficiently than they would otherwise be able to when handling large amounts of data.
For example, suppose you recently adopted 100 cats, which you allow to freely roam your 100-acre property. To figure out where and when to leave food for the cats, you equip 20 of them with GPS collars to track their movements throughout the day. Based on the data from the collars, you estimate where the best places to leave food are—you’ve used data to create a simple mathematical model that simulates the movement of your cats without having to track all 100 cats.
O’Neil argues that mathematical models are inherently reductive. Real-world events and people are immensely complex, and it’s impossible to input every factor that influences them into an equation. Lacking all the information necessary to simulate an event flawlessly, mathematical models inevitably produce a few mistakes. Organizations that use mathematical models are aware of these limitations, but they nonetheless use mathematical models because they’re willing to accept the trade-off between accuracy and efficiency.
To return to our example, your mathematical model of cat movements will occasionally return inaccurate results. As any cat owner can tell you, cats do what they want, when they want, coming and going in ways that aren’t entirely predictable. However, you decide that it’s still worth it to feed the cats based on the information your model spits out, as it’s much more efficient than tracking down and feeding each cat each day—and as long as all the cats are getting enough food, there’s no harm done.
O’Neil cautions that in addition to their limited accuracy, mathematical models also have ethical limitations. Mathematical models reflect the biases and incorrect assumptions of the people who make them. Additionally, unlike humans, mathematical models are unable to factor in moral and ethical concepts like equality.
For example, a cat feeding model designed by someone who incorrectly assumed that cats spend most of their lives in trees would probably place food sources up high. Based on this model, you’d end up distributing food unfairly, placing it where only the most nimble and adventurous cats would have access to it, while their terrestrial siblings would be left to fend for themselves.
The Characteristics of Dangerous Mathematical Models
Now that we understand what mathematical models are, let’s consider the three characteristics that make them dangerous: They’re opaque, they don’t incorporate feedback, and they operate on a large scale.
Dangerous Models Are Opaque
According to O’Neil, dangerous mathematical models don’t make their methods known to the public. Often, the inner workings of these models are considered valuable proprietary information—and the companies that own these models closely guard this information. O’Neil argues that this lack of transparency makes it impossible for outsiders to critique a particular model as outsiders aren’t permitted to know how the model works.
Lack of transparency also makes it impossible for individuals to protest the way models judge them. For example, if a bank uses a mathematical model to decide to deny you a loan and refuses to explain how the model reached that decision, you’re left with no recourse except to look elsewhere.
By contrast, good mathematical models make their methods transparent, says O’Neil. By publicizing the data and methods they use to make their judgments, they open themselves to critique—if a method is obviously flawed or unfair, transparency allows outsiders to point out the flaw so it can be corrected.
Transparency is especially important in models that judge individuals, such as credit scores. Transparency allows consumers to understand the criteria their score is based on and the steps they can take to improve their score.
Dangerous Models Don’t Incorporate Feedback
The next defining characteristic of dangerous mathematical models is a failure to incorporate feedback. According to O’Neil, good mathematical models are constantly incorporating feedback based on the accuracy of their predictions. If their predictions are accurate, the model remains unchanged, but when errors are detected, the model is adjusted. By incorporating feedback, good models slowly become more accurate over time, as their equations and algorithms are tweaked to avoid replicating old errors.
By contrast, dangerous models don’t collect or incorporate feedback. Models that don’t collect feedback remain oblivious to their own errors. If the original model includes bias, such models will continue to replicate that bias, without their designers ever realizing what’s happening.
Dangerous Models Operate on a Large Scale
The final characteristic of dangerous mathematical models is excessive scale. Good mathematical models are used at a reasonable scale, only in the contexts they were designed to simulate. When used within their designated contexts, good mathematical models often make accurate predictions, and when they fail, the harm they do is limited.
By contrast, dangerous mathematical models are deployed at massive scale, often far beyond the contexts they were originally designed for. O’Neil says that when used in such broad contexts, even low rates of inaccuracy cause harm to many people, as even small fractions of massive numbers often represent sizable groups of people.
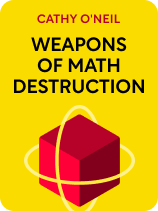
———End of Preview———
Like what you just read? Read the rest of the world's best book summary and analysis of Cathy O'Neil's "Weapons of Math Destruction" at Shortform.
Here's what you'll find in our full Weapons of Math Destruction summary:
- The insidious ways mathematical models are being used in society
- How mathematical models are being used to reinforce bias and harm the poor
- Recommendations for how to rein in dangerous mathematical models