
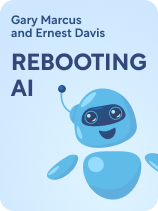
This article is an excerpt from the Shortform book guide to "Rebooting AI" by Gary Marcus and Ernest Davis. Shortform has the world's best summaries and analyses of books you should be reading.
Like this article? Sign up for a free trial here.
What’s the true potential of AI? What if AI could think and learn more like humans do? What would it take to get there?
In Rebooting AI, Gary Marcus and Ernest Davis explore the future of artificial intelligence. They argue that, to unlock the potential of AI, developers must create systems capable of human-level cognition. This entails drawing on research in neuroscience and psychology rather than relying solely on big data and current machine-learning techniques.
Keep reading to discover how strong AI could transform our world and why higher engineering standards are crucial for its development.
The Potential of AI
Marcus and Davis aren’t against AI—they simply believe that what the world needs is more research on strong AI development. The path to achieving strong AI systems that can genuinely understand and synthesize information requires drawing on more than big data and current machine learning techniques. The authors advocate for developers to explore the potential of AI by making use of current research in neuroscience and psychology to build systems capable of human-level cognition, ones that learn like the human brain does instead of merely correlating data. The authors add that these systems should be developed with more rigorous engineering standards than have been employed in the industry so far.
Davis and Marcus don’t deny that modern AI development has produced amazing advances in computing, but they state that we’re still falling short of AI’s true potential. An AI with the ability to understand data would be able to read all the research in a field—a task no human expert can do—while synthesizing that information to solve problems in medicine, economics, and the environment that stump even the brightest human minds. The advent of strong AI will be transformative for the whole human race, but Marcus and Davis insist that we won’t get there by feeding data to narrow AI systems. The AIs of the future will have to think and learn more like humans do, while being held to higher performance standards than modern AIs can achieve.
(Shortform note: Davis and Marcus’s assertion that strong AI should be modeled on the human brain goes back decades. In The Singularity Is Near, published in 2005, futurist Ray Kurzweil argues that creating a digital simulation of the brain is a necessary step in AI development. Kurzweil observes that the human brain’s major advantage over digital computers is that it’s massively parallel—it uses countless neural pathways operating in tandem, as opposed to traditional computing’s more linear approach. Mapping the brain’s multitude of parallel systems and simulating them in a digital environment may go a long way to addressing the issues that Marcus and Davis have with narrow AI.)
Human-Level Cognition
Narrow AIs are computational black boxes where information goes in, passes through a single (if convoluted) algorithm, then comes out reprocessed as a new result. That’s not how the human brain works, and Marcus and Davis argue that strong AI shouldn’t work like that either. Instead, AI research should draw on the efficiencies of the human brain, such as how it uses different processes for different types of information, how it creates abstract models of the world with which it interacts, and how it goes beyond correlating data to think in terms of causality—using mental models to understand how the external world changes over time.
Unlike narrow, single-system AI, the brain is a collection of systems that specialize in different types of information—sight, sound, and touch, for example—while regulating different forms of output, such as conscious and unconscious bodily functions. Likewise, Marcus and Davis write that strong AI should incorporate multiple processing systems and algorithms to handle the panoply of inputs and problems it will encounter in the real world. Also like the human brain, strong AI must be flexible enough to combine its different systems in whatever arrangement is needed at the moment, as humans do when we associate a memory with a smell, or when an artist engages both her visual and manual skills to produce a painting or a sculpture.
Mental Models and Causality
A more challenging but necessary step to strong AI will be designing knowledge frameworks that will let computers understand the relationships among entities, objects, and abstractions. These relations form the bedrock of human thought in the form of the mental models we use to interpret the world around us. Davis and Marcus state that modern AI developers largely disregard knowledge frameworks as an AI component. But without knowing how information interrelates, AI is unable to synthesize data from different sources and fields of knowledge. This ability is as crucial to advancing scientific progress as it is to driving a car in the snow while adjusting your schedule because of school closings—all of which AI could help with.
In addition to having a knowledge framework, strong AI must understand causality—how and why objects, people, and concepts change over time. Marcus and Davis say this will be hard to achieve because causality leads to correlation, but not all correlations are causal. For example, though most children like cookies (a correlation), enjoying cookies doesn’t cause childhood. Furthermore, AI will have to juggle causality in multiple fields of knowledge at once. For instance, an AI working on a legal case will have to understand how human motivations interact with physical evidence. At present, the only way for a computer to model causality is to run multiple simulations, but that’s far less efficient than how the human brain works. Therefore, we must design our strong AIs to learn these concepts the way that humans learn.
Brain-Like Learning
When it comes to teaching computers how to think, we can draw from millions of years of human evolution and incorporate facets of the human brain’s tried-and-true approach to learning into machine cognition. While current machine learning operates from a starting point of pure data, Davis and Marcus argue that preprogrammed knowledge about the world similar to what humans are born with can facilitate stronger AI development. The authors describe how the brain learns by combining first-hand experience with preexisting knowledge, how AIs could use this tactic to construct their own knowledge frameworks, and why a hybrid approach to machine learning would be more powerful than the current data-only techniques.
When learning, people draw from two sources—high-level conceptualizations that are either instinctive or taught to us by others, and low-level details that we absorb through day-to-day experiences. Our preexisting, high-level knowledge provides a vital framework through which we interpret whatever we discover on our own. For example, we’re born knowing that food goes into our mouths and tastes good—as children, we then use this framework to determine what does and doesn’t qualify as food. However, Marcus and Davis report that AI developers shun the idea of preprogramming knowledge into neural networks, preferring their systems to learn from data alone, free of any context that would help it make sense.
Preprogrammed knowledge frameworks—like a set of “instincts” an AI would be born with— could greatly advance AI language comprehension. When humans read or listen to language, we construct a mental model of what’s being described based on our prior understanding of the world. Davis and Marcus argue that giving an AI a preprogrammed basis for connecting language to meaning would let it construct its own knowledge frameworks, just as humans learn over time. By insisting that AIs learn from raw data alone, developers tie their hands behind their backs and create an impossible task for AI, like giving a calculator the complete works of Shakespeare and expecting it to deduce the English language from scratch.
Marcus and Davis conclude that neither preprogrammed knowledge nor enormous data dumps are sufficient in themselves to teach computers how to think. The road to strong AI will require a combination of engineered cognitive models and large amounts of input data so that artificial intelligence can have a fighting chance to train itself. Knowledge frameworks can give AI the capability for logic and reason beyond their current parlor tricks of generating output from statistical correlations. Meanwhile, absorbing information from big data can give AI the experience to build its own cognitive models, growing beyond its programmers’ designs.
Higher Engineering Standards
As AI permeates our lives more and more, the degree to which it functions well or poorly will have more of an impact on the world. However, because many AI applications have been in fields like advertising and entertainment where the human consequences of error are slight, AI developers have grown lackadaisical about performance standards. Davis and Marcus discuss their AI safety concerns, the difficulty of measuring an AI’s performance, and the minimum expectations we should have regarding AI’s reliability before we hand over the reins of power.
In most industries, engineers design systems to withstand higher stressors than they’re likely to encounter in everyday use, with backup systems put in place should anything vital to health and safety fail. Marcus and Davis say that compared to other industries, software development has a much lower bar for what counts as good performance. This already manifests as vulnerabilities in our global information infrastructure. Once we start to put other vital systems in the hands of unreliable narrow AI, a slipshod approach to safety and performance could very well have disastrous consequences, much more so than chatbot hallucinations.
Exacerbating AI’s issues with performance, when AIs go wrong, they’re very hard to debug precisely because of how neural networks work. For this reason, Davis and Marcus are engaged in research on ways to measure AI’s progress and performance. One method they hope to adapt for AI is “program verification”—an approach that’s been used in classical software to confirm that a program’s outputs match expectations. They also recommend that other AI designers explore a similar approach to improving performance, perhaps by using comparable AI systems to monitor each other’s functionality.
It would be unrealistic to expect any computer system to be perfect. However, the weakness of narrow AI is that without human-level comprehension, it’s prone to unpredictable, nonsensical errors outside the bounds of merely human mistakes. Marcus and Davis insist that until we develop stronger AI systems, people should be careful not to project human values and understanding on these purely automated systems. Most of all, if we’re to grant AI increasing levels of control, we should demand that AI have the same shared understanding of the world that we’d expect from our fellow human beings.
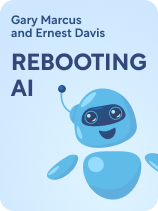
———End of Preview———
Like what you just read? Read the rest of the world's best book summary and analysis of Gary Marcus and Ernest Davis's "Rebooting AI" at Shortform.
Here's what you'll find in our full Rebooting AI summary:
- That AI proponents oversell what modern AI can accomplish
- How AI in its current form underdelivers on its creators’ promises
- The real danger—and promise—of artificial intelligence