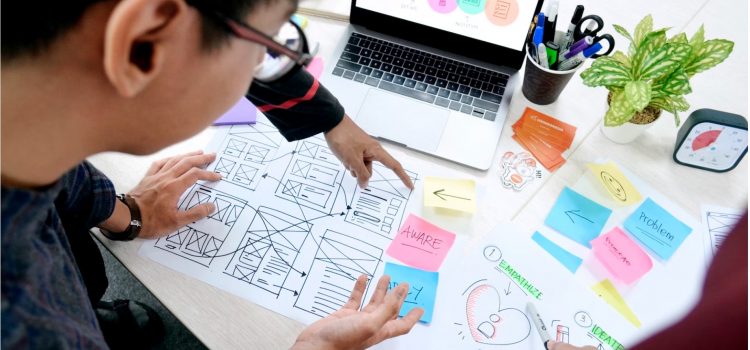
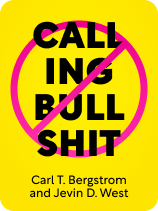
This article is an excerpt from the Shortform book guide to "Calling Bullshit" by Carl T. Bergstrom and Jevin D. West. Shortform has the world's best summaries and analyses of books you should be reading.
Like this article? Sign up for a free trial here.
Should you trust data-based arguments? How can data go terribly wrong?
In Calling Bullshit, Carl T. Bergstrom and Jevin D. West investigate how bullshit is created. They assert that it happens when people use faulty data as a basis for their arguments. Specifically, they say selection bias can lead to bullshit because it justifies faulty conclusions based on unrepresentative samples.
Read more to understand how selection bias in statistics can lead to harmful misinformation.
Selection Bias in Statistics
Bergstrom and West explain that selection bias in statistics occurs whenever the population sampled for a research study doesn’t represent the broader population that you’re interested in. For example, if you wanted to know how the US population would vote in the 2024 election, you could commit selection bias by only polling, say, senior citizens. And, although selection bias takes many forms, Bergstrom and West focus on two in particular: the observation selection effect and data censoring.
(Shortform note: Experts note that selection bias is prevalent in political polling, where convenience sampling—the practice of polling individuals by a convenient method that isn’t random—often skews the results of polls. Even in modern polls, this remains a problem. In 2012, for example, experts say polls by the polling company Rasmussen consistently, but incorrectly, predicted Republican candidate Mitt Romney to win the popular vote over Democratic candidate Barack Obama in the US elections. According to these experts, this might have occurred because Rasmussen contacts individuals via landline phones and online polls, both of which are slightly biased toward wealthier voters more likely to vote Republican.)
Selection Bias #1: The Observation Selection Effect
Bergstrom and West maintain that selection bias can creep into data via the observation selection effect, which occurs when our data collection process is correlated with the variable that we’re collecting data on. For example, if we could only conduct presidential polls via smartphones, the correlation between smartphone ownership and presidential preference would undermine the integrity of these polls—smartphone owners may be wealthier, on average, and therefore more likely to vote for a candidate who cuts taxes for the rich.
(Shortform note: The observation selection effect causes notorious issues in philosophy and science, where the fact that we exist to observe certain phenomena presupposes certain conditions about the universe. For example, some philosophers take the observation that the universe is surprisingly hospitable to sentient life as evidence of a creator, even though this hospitability is necessary for us to observe it in the first place.)
The observation selection effect, Bergstrom and West argue, can yield misleading conclusions that bullshitters can prop up. Returning to the previous example, bullshitters could use poll data conducted exclusively by smartphone to spread a false narrative that one candidate was guaranteed to win, when in fact their chances might be much lower than the poll suggests.
(Shortform note: In the case of polling, one reason bullshitters might want to use biased polls is that such polls can influence elections via the contagion effect—the tendency of voters to rally around candidates who are polling well. For example, if a misleading poll suggested that one candidate was crushing the other candidates in a primary election, that could cause more voters to view that candidate’s victory as inevitable and therefore vote for them.)
Selection Bias #2: Data Censoring
Bergstrom and West explain that in addition to the observation selection effect, data censoring can be another source of selection bias in statistics. They relate that data censoring occurs whenever an initially random sample becomes non-random at the completion of a study because a non-random subset of that initial sample was ineligible for inclusion in the study’s results.
For instance, imagine that we conducted a study in 2023 assessing the life expectancy of individuals born in the 1900s versus those born in the 2000s. Because individuals who are still alive can’t figure into our results, the 2000s sample would appear to have a drastically lower life expectancy because it could only include those who had died by 2023 (and thus were at most 23 years old). Although data censoring is rare, Bergstrom and West caution that its misleading results can be propagated by bullshitters.
(Shortform note: A close relative of data censoring is truncated data, which occurs whenever observations are systematically excluded from our data set. For example, if we were testing the relationship between someone’s age and their response to a given antibiotic, we could truncate the data by not considering anyone below the age of 25 in our study. So, when the truncated data are relevant to the study’s conclusions, truncation can be a means to promote bullshit.)
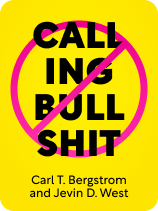
———End of Preview———
Like what you just read? Read the rest of the world's best book summary and analysis of Carl T. Bergstrom and Jevin D. West's "Calling Bullshit" at Shortform.
Here's what you'll find in our full Calling Bullshit summary:
- That misinformation online, in news, and in academia is spreading
- How to detect and refute bullshit in its many forms
- How data can be miscollected and misinterpreted in science