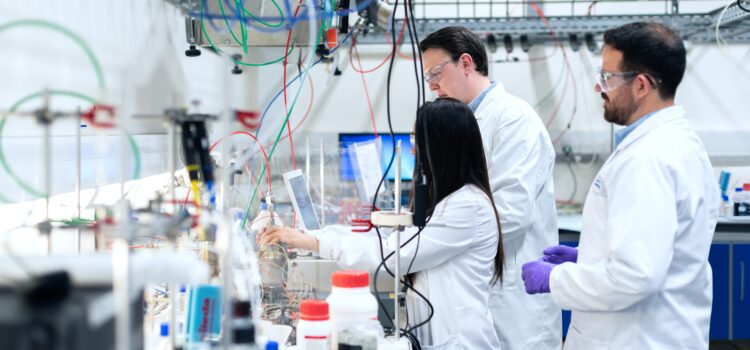
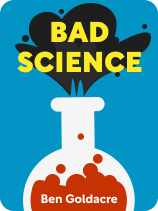
This article is an excerpt from the Shortform book guide to "Bad Science" by Ben Goldacre. Shortform has the world's best summaries and analyses of books you should be reading.
Like this article? Sign up for a free trial here.
What is selection bias in research? How does it affect scientific findings?
Selection bias is when researchers sort participants into different groups to get their desired result. According to Ben Goldacre in Bad Science, this unethical tactic can cause a systematic error that could’ve been avoided with randomization.
Let’s look at why selection bias in research is a growing issue.
Randomization and Researcher Bias
Goldacre describes how unscrupulous researchers manipulate results by strategically sorting participants into different groups, also known as selection bias in research. For instance, if you wanted to make your new drug seem more effective than it is, you could decide to give your drug to the patients who were healthiest and most likely to improve anyway, while giving the standard treatment to the patients in the worst condition.
(Shortform note: Scientists refer to the influence of poor selection and sorting methods as selection bias. Experts note that even when researchers don’t intentionally manipulate their trials, weak methods can allow selection bias to affect a trial’s results.)
To prevent these kinds of manipulations, scientists use a process called randomization. Goldacre describes randomization as the process of randomly determining which treatment a patient receives. By assigning participants randomly, you can prevent any one group from unfairly receiving only the participants that are most likely to succeed.
(Shortform note: Randomization has many additional benefits. Researchers argue that randomized trials are especially useful for determining causal relationships. This is because randomized trials ensure that the only differences between different groups are the treatments they receive. As a result, any differences in the results of different groups can be confidently chalked up to the treatment each received.)
Spotting Bad Randomization
There are a few different shady randomization practices to look out for when evaluating research. Specifically, Goldacre is skeptical of the practice of assigning treatments based on the order that participants sign up for the trial. In a system like this, the first 10 participants to sign up might get treatment A, while the second group of 10 all get treatment B, and so on. While on the surface, this procedure might seem random, it leaves a lot of room for recruiters to influence results. For example, if you know that the next patient you recruit will be placed into the group testing your new treatment, you might choose a patient who you think is likely to get well anyway—when they do, it’ll appear as though your treatment worked.
(Shortform note: In addition to looking out for randomization methods based on signup order, you should also ask yourself if each group of participants is large and varied enough to accurately generalize to larger populations. For example, a study with only five participants is unlikely to contain enough variety to be compared to larger populations. However, a study with several thousand participants is more likely to produce more comprehensive findings.)
Goldacre notes that some researchers manipulate their studies by randomizing patients based on factors that more subtly affect results. For instance, suppose you come up with a randomization strategy that sorts participants into groups based on ZIP code. While this seems random, some ZIP codes might contain more affluent neighborhoods, where residents can generally afford better health care. If these more affluent patients were all sorted into the same treatment group, it might make that treatment seem more effective than it really is.
(Shortform note: These kinds of differences between the general population and the participant group used in an experiment are known as random sampling error. All studies include some degree of sampling error. While in our example the sampling error was due to intentional manipulation, sampling error is often a natural consequence of an experiment’s logistical limitations—no group of people small enough to study will ever be perfectly representative of the world’s population.)
By contrast, good randomization methods have little room for bias to influence results. It’s a good sign when researchers describe keeping their recruiting processes and their treatment assignment processes separate. This prevents recruiter bias from interfering with randomization. Additionally, Goldacre states that studies that use randomization software are also likely to be trustworthy—using computers for randomization reduces the effects of human bias and error.
(Shortform note: One issue that makes it hard to judge whether randomization methods have affected a study is that even strong randomization practices sometimes produce unbalanced groups. For example, suppose you flip a coin five times in a row. While it’s unlikely, if you did this enough times, you’d eventually get heads five times in a row. To account for unlucky failures in randomization, as well as other factors, the scientific method emphasizes reproducibility. Generally, you should only consider a trial’s results to be reliable if researchers can reproduce the same result when conducting the experiment a second time.)
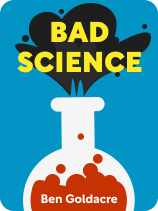
———End of Preview———
Like what you just read? Read the rest of the world's best book summary and analysis of Ben Goldacre's "Bad Science" at Shortform.
Here's what you'll find in our full Bad Science summary:
- The strategies researchers, corporations, and journalists use to mislead the public
- The tools you need to identify and call out shady science when you see it
- Why media outlets have an ethical obligation to publish retractions