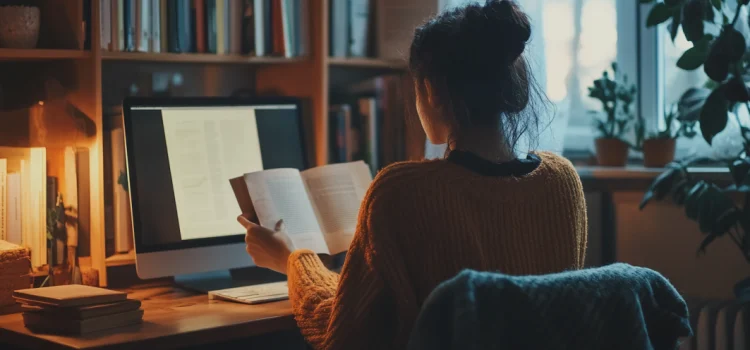
Is AI a cornucopia that could free the human race from drudgery? Or will AI bring about the end of the world as we know it?
Artificial Intelligence (AI) has the potential to revolutionize every aspect of the way we live. AI’s future applications could be enormous—but only if we can develop machine intelligence that can accurately and reliably carry out its tasks.
Read on for an overview of Rebooting AI: Building Artificial Intelligence We Can Trust by Gary Marcus and Ernest Davis.
Overview of Rebooting AI
In Rebooting AI: Building Artificial Intelligence We Can Trust, published in 2019, Gary Marcus and Ernest Davis argue that AI proponents oversell what modern AI can accomplish, while AI in its current form underdelivers on its creators’ promises. Marcus and Davis also suggest that those who fear an AI takeover are worried about the wrong thing. The danger isn’t that an evil AI will conquer the world, but that we’ll cede power to unreliable systems which, through their lack of real-world comprehension, are likely to endanger people’s lives by making idiotic mistakes no human could conceive of.
Marcus and Davis are AI advocates, who nevertheless feel that current research on AI development is heading down a dead-end street, with its narrow focus on “big data” and machine learning to the exclusion of other important tools. Marcus has a Ph.D. in psychology, is the founder of the companies Geometric Intelligence and Robust.AI, and has authored several books on learning and cognition, including Kluge and Guitar Zero. Davis is a professor of computer science at New York University and has written previous works on artificial intelligence, including Representations of Commonsense Knowledge.
Don’t Believe the Hype About AI
Before discussing whether AI works or doesn’t, Marcus and Davis address how public perception of artificial intelligence is skewed. The computer industry, science fiction, and the media have primed the public to imagine “strong AI”—computer systems that actually think and can do so much faster and more powerfully than humans. Instead, what AI developers have delivered is “narrow AI”—systems trained to do one specific task while having no more awareness of the larger world than a doorknob can understand what a door is for. The authors explain how AI’s capabilities are currently being oversold and why software engineers and the public at large are susceptible to overestimating narrow AI’s capabilities.
AI Fantasy Versus Reality
The AI systems making an impact today are far from the benevolent androids or evil computer overlords depicted in science fiction. Instead, Davis and Marcus describe modern AIs as hyper-focused morons who don’t understand that a world exists beyond the tasks they’re trained on. Yet the public’s confusion is understandable—tech companies like to garner attention by billing every incremental step forward as a giant leap for AI, while the press eagerly overhypes AI progress in search of more readers and social media clicks.
To be fair, technology has made great advances in using big data to drive machine learning. Marcus and Davis take care to point out that we haven’t built machines that understand the data they use and adapt to real-world settings, and the authors list some reasons why this is sometimes hard to see. The first is our tendency to project human qualities on objects that don’t have them—such as claiming that your car gets “cranky” in cold weather, or imagining that your house’s bad plumbing has a vendetta against you. When a computer does things that were once uniquely human, such as answering a question or giving you driving directions, it’s easy to mistake digital abilities for actual thought.
Shortsightedness in AI Development
The second reason that Davis and Marcus say that modern AI’s abilities are overstated is that developers tend to mistakenly believe that progress on small computing challenges equates to progress toward larger goals. Much of the work on narrow AI has been done in environments with clear rules and boundaries, such as teaching a computer to play chess or a robot to climb a set of stairs. Unfortunately, these situations don’t map onto the real world, where the number of variables and shifting conditions are essentially infinite. No matter how many obstacles you teach a stair-climbing robot to avoid under laboratory conditions, that can never match the infinitude of problems it might stumble upon in an everyday house.
So far, according to Marcus and Davis, AI developers have largely ignored the issue of how to train AI to cope in situations it’s not programmed for, such as setting the stair-climbing robot’s stairs on fire. This is because, for most of its history, artificial intelligence has been used in situations where the cost of failure is usually low, such as recommending books based on your reading history or calculating the best route to work based on current traffic conditions. The authors warn that if we start to use narrow AI in situations where the cost of failure is high, it will put people’s health and safety at risk in ways that no one will be able to predict.
How Narrow AI Works
To understand Davis and Marcus’s concerns about narrow AI, it’s important to grasp how narrow AI operates. The current paradigm of AI development combines artificial “neural networks” that can be trained to produce desired outputs when they’re fed vast amounts of data. The authors describe how this process developed, the way that it permeates—and benefits—modern technology, and what it lacks in terms of reaching true intelligence.
In the 20th century, software developers had to manually input the instructions for anything a computer had to do. When it came to simulating intelligence, this process was unfeasible, so researchers developed the first neural networks that could perform “intelligent” tasks by analyzing large amounts of data. This was also a cumbersome approach until the early 2010s, when computer processors grew fast enough to cope with the huge datasets that became available. Neural networks became deeper and deeper—meaning more computational layers could be added between inputs such as queries and the computer’s output.
Power in Statistics
Marcus and Davis explain that, despite its complexity, machine learning relies entirely on statistical probabilities to determine its outputs. Nevertheless, it’s now found everywhere, mostly to positive results, from the system that recommends movies on your streaming service to the facial recognition software used in airport security. Modern search engines wouldn’t work at all without big data and machine learning, nor would many of the programs we now use, which can be “taught” to computers much quicker than they could ever be keyed in by hand. Thanks to these successes, neural networks and machine learning are the driving force behind AI development as the industry presently stands.
Nevertheless, Davis and Marcus insist that machine learning has one major flaw—statistical correlation doesn’t lead to true intelligence; it can only create the illusion of understanding. Some developers have tried to overcome this hurdle by leveraging the human understanding of their users. For instance, the software behind social media sites learns to prioritize links and posts that human users click on more often. When that strategy leads to poor results, such as perpetuating bias or misleading information, engineers try to solve it problem-by-problem without addressing the underlying issue—that the AI running the social media site has no real comprehension of what the information it’s spreading really means.
How Narrow AI Fails
So far, AIs have been successful when they’ve been designed to do a single task. Their problem is reliability—even if they work most of the time, we never know when they’ll make nonsensical mistakes that no human ever would. Marcus and Davis blame AI’s problems on fundamental design deficiencies, including issues with how machine learning works, the nature of the data it’s trained on, problems with how computers process language, and the way that machines perceive the physical world.
Problems With Machine Learning and Data
Davis and Marcus’s main objection to training neural networks using large amounts of data is that, when this strategy is employed to the exclusion of every other programming tool, it’s hard to correct for a system’s dependence on statistical correlation instead of logic and reason. Because of this, neural networks can’t be debugged in the way that human-written software can, and they’re easily fooled when presented with data that don’t match what they’re trained on.
AI Hallucinations
When neural networks are solely trained on input data rather than programmed by hand, it’s impossible to say exactly why the system produces a particular result from any given input. For example, suppose an airport computer trained to identify approaching aircraft mistakes a flight of geese for a Boeing 747. In AI development, this kind of mismatch error is referred to as a “hallucination,” and, under the wrong circumstances—such as in an airport control tower—the disruptions an AI hallucination might cause range from costly to catastrophic.
Marcus and Davis write that, when AI hallucinations occur, it’s impossible to identify where the errors take place in the maze of computations inside a neural network. This makes traditional debugging impossible, so software engineers have to “retrain” that specific error out of the system, such as by giving the airport computer’s AI thousands of photos of birds in flight that are clearly labeled as “not airplanes.” Davis and Marcus argue that this solution does nothing to fix the systemic issues that cause hallucinations in the first place.
Hallucinations and Big Data
AI hallucinations aren’t hard to produce, as anyone who’s used ChatGPT can attest. In many cases, AIs hallucinate when presented with information in an unusual context that’s not similar to what’s included in the system’s training data. Consider the popular YouTube video of a cat dressed as a shark riding a Roomba. No matter that the image is bizarre, a human has no difficulty identifying what they’re looking at, whereas an AI tasked with the same assignment would offer a completely wrong answer. Davis and Marcus argue that this matters when pattern recognition is used in critical situations, such as in self-driving cars. If the AI scanning the road ahead sees an unusual object in its path, the system could hallucinate with disastrous results.
Hallucinations illustrate a difference between human and machine cognition—we can make decisions based on minimal information, whereas machine learning requires huge datasets to function. Marcus and Davis point out that, if AI is to interact with the real world’s infinite variables and possibilities, there isn’t a big enough dataset in existence to train an AI for every situation. Since AIs don’t understand what their data mean, only how those data correlate, AIs will perpetuate and amplify human biases that are buried in their input information. There’s a further danger that AI will magnify its own hallucinations as erroneous computer-generated information becomes part of the global set of data used to train future AI.
Problems With Language
A great deal of AI research has focused on systems that can analyze and respond to human language. While the development of language interfaces has been a vast benefit to human society, Davis and Marcus insist that the current machine language learning models leave much to be desired. They highlight how language systems based entirely on statistical correlations can fail at even the simplest of tasks and why the ambiguity of natural speech is an insurmountable barrier for the current AI paradigm.
It’s easy to imagine that, when we talk to Siri or Alexa, or phrase a search engine request as an actual question, that the computer understands what we’re asking; but Marcus and Davis remind us that AIs have no idea that words stand for things in the real world. Instead, AIs merely compare what you say to a huge database of preexisting text to determine the most likely response. For simple questions, this tends to work—but if your phrasing of a request doesn’t match the AI’s database, the odds of it not responding correctly increase. For instance, if you ask Google “How long has Elon Musk been alive?” it will tell you the date of his birth but not his age unless that data is spelled out online in something close to the way you asked it.
A Deficiency of Meaning
Davis and Marcus say that, though progress has been made in teaching computers to differentiate parts of speech and basic sentence structure, AIs are unable to compute the meaning of a sentence from the meaning of its parts. As an example, ask a search engine to “find the nearest department store that isn’t Macy’s.” What you’re likely to get is a list of all the Macy’s department stores in your area, clearly showing that the search engine doesn’t understand how the word “isn’t” relates to the rest of the sentence.
An even greater difficulty arises from the inherent ambiguity of natural language. Many words have multiple meanings, and sentences take many grammatical forms. However, Marcus and Davis illustrate that what’s most perplexing to AI are the unspoken assumptions behind every human question or statement. Given their limitations, no modern AI can read between the lines. Every human conversation rests on a shared understanding of the world that both parties take for granted, such as the patterns of everyday life or the basic laws of physics that constrain how we behave. Since AI language models are limited to words alone, they can’t understand the larger reality that words reflect.
Problems With Awareness
While language-processing AI ignores the wider world, mapping and modeling objects in space is of primary concern in the world of robotics. Since robots and the AIs that run them function in the physical realm beyond the safety of mere circuit pathways, they must observe and adapt to their physical surroundings. Davis and Marcus describe how both traditional programming and machine learning are inadequate for this task, and they explore why future AIs will need a broader set of capabilities than we currently have in development if they’re to safely navigate the real world.
Robots are hardly anything new—we’ve had robotic machinery in factories for decades, not to mention the robotic space probes we’ve sent to other planets. However, all our robots are limited in function and the range of environments in which they operate. Before neural networks, these machines were either directly controlled or programmed by humans to behave in certain ways under specific conditions to carry out their tasks and avoid a limited number of foreseeable obstacles.
Real-World Complexity
With machine learning’s growing aptitude for pattern recognition, neural network AIs operating in the real world are getting better at classifying the objects that they see. However, statistics-based machine learning systems still can’t grasp how real-world objects relate to each other, nor can AIs fathom how their environment can change from moment to moment. Researchers have made progress on the latter issue using video game simulations, but Marcus and Davis argue that an AI-driven robot in the real world could never have time to simulate every possible course of action. If someone steps in front of your self-driving car, you don’t want the car to waste too much time simulating the best way to avoid them.
Davis and Marcus believe that an AI capable of safely interacting with the physical world must have a human-level understanding of how the world actually works. It must have a solid awareness of its surroundings, the ability to plan a course of action on its own, and the mental flexibility to adjust its behavior on the fly as things change. In short, any AI whose actions and decisions are going to have real-world consequences beyond the limited scope of one task needs to be strong AI, not the narrow AIs we’re developing now.
The Road to Strong AI
To be clear, Marcus and Davis aren’t against AI—they simply believe that what the world needs is more research on strong AI development. The path to achieving strong AI systems that can genuinely understand and synthesize information requires drawing on more than big data and current machine learning techniques. The authors advocate for AI developers to make use of current research in neuroscience and psychology to build systems capable of human-level cognition, ones that learn like the human brain does instead of merely correlating data. The authors add that these systems should be developed with more rigorous engineering standards than have been employed in the industry so far.
Davis and Marcus don’t deny that modern AI development has produced amazing advances in computing, but they state that we’re still falling short of AI’s true potential. An AI with the ability to understand data would be able to read all the research in a field—a task no human expert can do—while synthesizing that information to solve problems in medicine, economics, and the environment that stump even the brightest human minds. The advent of strong AI will be transformative for the whole human race, but Marcus and Davis insist that we won’t get there by feeding data to narrow AI systems. The AIs of the future will have to think and learn more like humans do, while being held to higher performance standards than modern AIs can achieve.
Human-Level Cognition
Narrow AIs are computational black boxes where information goes in, passes through a single (if convoluted) algorithm, then comes out reprocessed as a new result. That’s not how the human brain works, and Marcus and Davis argue that strong AI shouldn’t work like that either. Instead, AI research should draw on the efficiencies of the human brain, such as how it uses different processes for different types of information, how it creates abstract models of the world with which it interacts, and how it goes beyond correlating data to think in terms of causality—using mental models to understand how the external world changes over time.
Unlike narrow, single-system AI, the brain is a collection of systems that specialize in different types of information—sight, sound, and touch, for example—while regulating different forms of output, such as conscious and unconscious bodily functions. Likewise, Marcus and Davis write that strong AI should incorporate multiple processing systems and algorithms to handle the panoply of inputs and problems it will encounter in the real world. Also like the human brain, strong AI must be flexible enough to combine its different systems in whatever arrangement is needed at the moment, as humans do when we associate a memory with a smell, or when an artist engages both her visual and manual skills to produce a painting or a sculpture.
Mental Models and Causality
A more challenging but necessary step to strong AI will be designing knowledge frameworks that will let computers understand the relationships among entities, objects, and abstractions. These relations form the bedrock of human thought in the form of the mental models we use to interpret the world around us. Davis and Marcus state that modern AI developers largely disregard knowledge frameworks as an AI component. But without knowing how information interrelates, AI is unable to synthesize data from different sources and fields of knowledge. This ability is as crucial to advancing scientific progress as it is to driving a car in the snow while adjusting your schedule because of school closings—all of which AI could help with.
In addition to having a knowledge framework, strong AI must understand causality—how and why objects, people, and concepts change over time. Marcus and Davis say this will be hard to achieve because causality leads to correlation, but not all correlations are causal. For example, though most children like cookies (a correlation), enjoying cookies doesn’t cause childhood. Furthermore, AI will have to juggle causality in multiple fields of knowledge at once. For instance, an AI working on a legal case will have to understand how human motivations interact with physical evidence. At present, the only way for a computer to model causality is to run multiple simulations, but that’s far less efficient than how the human brain works. Therefore, we must design our strong AIs to learn these concepts the way that humans learn.
Brain-Like Learning
When it comes to teaching computers how to think, we can draw from millions of years of human evolution and incorporate facets of the human brain’s tried-and-true approach to learning into machine cognition. While current machine learning operates from a starting point of pure data, Davis and Marcus argue that preprogrammed knowledge about the world similar to what humans are born with can facilitate stronger AI development. The authors describe how the brain learns by combining first-hand experience with preexisting knowledge, how AIs could use this tactic to construct their own knowledge frameworks, and why a hybrid approach to machine learning would be more powerful than the current data-only techniques.
When learning, people draw from two sources—high-level conceptualizations that are either instinctive or taught to us by others, and low-level details that we absorb through day-to-day experiences. Our preexisting, high-level knowledge provides a vital framework through which we interpret whatever we discover on our own. For example, we’re born knowing that food goes into our mouths and tastes good—as children, we then use this framework to determine what does and doesn’t qualify as food. However, Marcus and Davis report that AI developers shun the idea of preprogramming knowledge into neural networks, preferring their systems to learn from data alone, free of any context that would help it make sense.
Preprogrammed knowledge frameworks—like a set of “instincts” an AI would be born with— could greatly advance AI language comprehension. When humans read or listen to language, we construct a mental model of what’s being described based on our prior understanding of the world. Davis and Marcus argue that giving an AI a preprogrammed basis for connecting language to meaning would let it construct its own knowledge frameworks, just as humans learn over time. By insisting that AIs learn from raw data alone, developers tie their hands behind their backs and create an impossible task for AI, like giving a calculator the complete works of Shakespeare and expecting it to deduce the English language from scratch.
Marcus and Davis conclude that neither preprogrammed knowledge nor enormous data dumps are sufficient in themselves to teach computers how to think. The road to strong AI will require a combination of engineered cognitive models and large amounts of input data so that artificial intelligence can have a fighting chance to train itself. Knowledge frameworks can give AI the capability for logic and reason beyond their current parlor tricks of generating output from statistical correlations. Meanwhile, absorbing information from big data can give AI the experience to build its own cognitive models, growing beyond its programmers’ designs.
Higher Engineering Standards
As AI permeates our lives more and more, the degree to which it functions well or poorly will have more of an impact on the world. However, because many AI applications have been in fields like advertising and entertainment where the human consequences of error are slight, AI developers have grown lackadaisical about performance standards. Davis and Marcus discuss their AI safety concerns, the difficulty of measuring an AI’s performance, and the minimum expectations we should have regarding AI’s reliability before we hand over the reins of power.
In most industries, engineers design systems to withstand higher stressors than they’re likely to encounter in everyday use, with backup systems put in place should anything vital to health and safety fail. Marcus and Davis say that compared to other industries, software development has a much lower bar for what counts as good performance. This already manifests as vulnerabilities in our global information infrastructure. Once we start to put other vital systems in the hands of unreliable narrow AI, a slipshod approach to safety and performance could very well have disastrous consequences, much more so than chatbot hallucinations.
Exacerbating AI’s issues with performance, when AIs go wrong, they’re very hard to debug precisely because of how neural networks work. For this reason, Davis and Marcus are engaged in research on ways to measure AI’s progress and performance. One method they hope to adapt for AI is “program verification”—an approach that’s been used in classical software to confirm that a program’s outputs match expectations. They also recommend that other AI designers explore a similar approach to improving performance, perhaps by using comparable AI systems to monitor each other’s functionality.
It would be unrealistic to expect any computer system to be perfect. However, the weakness of narrow AI is that without human-level comprehension, it’s prone to unpredictable, nonsensical errors outside the bounds of merely human mistakes. Marcus and Davis insist that until we develop stronger AI systems, people should be careful not to project human values and understanding on these purely automated systems. Most of all, if we’re to grant AI increasing levels of control, we should demand that AI have the same shared understanding of the world that we’d expect from our fellow human beings.