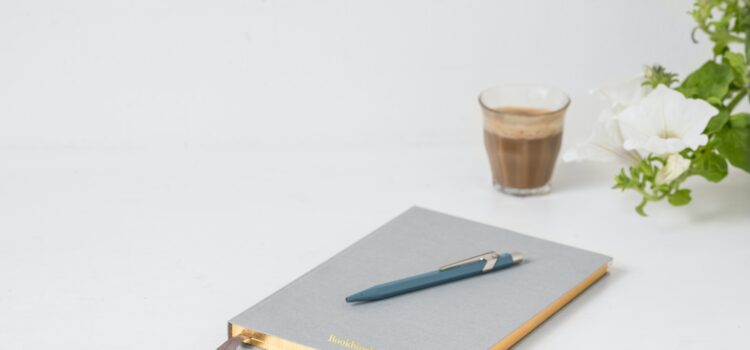
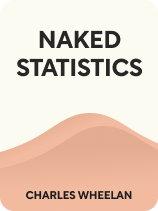
This article is an excerpt from the Shortform book guide to "Naked Statistics" by Charles Wheelan. Shortform has the world's best summaries and analyses of books you should be reading.
Like this article? Sign up for a free trial here .
What is the null hypothesis in statistics? What does accepting a null hypothesis tell us about the relationship between two variables?
When we use inferential statistics to answer a question, we begin with a null hypothesis. A null hypothesis assumes a relationship between two variables that we’ll accept or reject. If the null hypothesis is rejected, we accept the alternative hypothesis, which is the logical inverse of the null hypothesis.
Keep reading to learn about the null hypothesis, why we use it, and what accepting/rejecting it can tell us about data.
The Null and Alternative Hypotheses
In inferential statistics, the convention is to begin with a null hypothesis that we hope or expect to reject. For example, a vitamin company might hope to reject the null hypothesis that absorption of their new vitamin is no better than absorption of their previous formula.
Rejecting a null hypothesis means “accepting” an alternative hypothesis. For example, if the vitamin company’s null hypothesis is that absorption of their new vitamin is no better than absorption of their previous formula, their alternative hypothesis is that absorption of their new vitamin is better than absorption of their previous formula.
Why a Null Hypothesis?
The convention of accepting or rejecting a null hypothesis in statistics might seem like a redundant step. However, this convention upholds a fundamental rule in science: You can only disprove hypotheses, never prove them.
For example, say your hypothesis is that a certain species of bear only eats meat because that is all you have ever observed this type of bear eating. You can’t possibly collect data on every individual bear of that species and everything they have ever eaten to prove your hypothesis. However, if you observe one of the bears eating berries, your hypothesis is quickly disproven.
While we can’t prove anything in science, we can collect data to support a hypothesis, which is the role of the alternative hypothesis. Therefore, the null hypothesis provides the opportunity to disprove an idea, and the alternative hypothesis provides the opportunity to use the results of our calculations to support an alternative idea.
Criticism of the Null Hypothesis Approach
The null hypothesis system provides a systematic and standardized approach to assessing data with statistics. However, it’s also criticized for allowing “sloppy” scientific research to pass as acceptable. We’ll highlight two of these critiques.
The convention of accepting or rejecting a null hypothesis as the final step in data analysis is a suboptimal way to further scientific knowledge. First, when the null hypothesis is accepted, data from these “negative findings” are often not published or shared with the scientific community or public. This leads to an underrepresentation of negative results in the scientific literature and an overrepresentation of positive results.
For example, 10 studies could show no link between eating a certain food and getting arthritis. But if one study did show a statistically significant relationship between the two, it would be published and shape public perception. The still-valuable data from the nine negative findings would likely be forgotten.
The fact that statistical analyses are reported with a certain confidence level is another reason to include data from negative findings in the scientific literature. Even the highest confidence levels leave room for Type ll errors (discussed below), meaning statistically significant findings are reported as not significant. This means there is likely a great deal of important and statistically significant research that goes unread by the scientific community.
Second, when the goal of data analysis is to reject the null hypothesis, too little emphasis is placed on the alternative hypothesis. Since the convention when rejecting the null hypothesis is to accept the alternative hypothesis, the alternative hypothesis should also be well-researched and thought out. However, it’s often simply presented as the opposite of the null hypothesis.
For example, if you rejected my null hypothesis that there is no relationship between eating a certain food and arthritis, and accepted the alternative hypothesis that there is a relationship between eating a certain food and arthritis, that might be the end of the analysis in your publication. However, this leaves your readers with no context for what the relationship between food and arthritis is. Therefore, an alternative hypothesis based solely on the rejection of the null hypothesis can lead to nebulous conclusions that are less informative than ideas making their way into scientific publications “should” be.
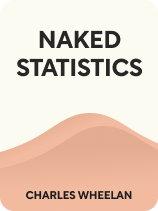
———End of Preview———
Like what you just read? Read the rest of the world's best book summary and analysis of Charles Wheelan's "Naked Statistics" at Shortform .
Here's what you'll find in our full Naked Statistics summary :
- An explanation and breakdown of statistics into digestible terms
- How statistics can inform collective decision-making
- Why learning statistics is an exercise in self-empowerment