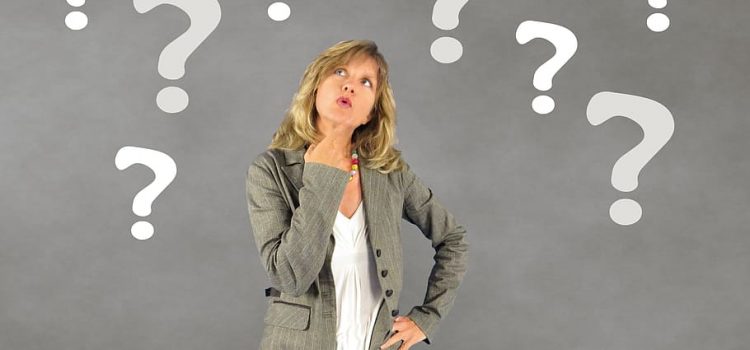
Why do we make decisions that contradict basic logic? What are some examples of illogical decision-making?
One of the most common reasons we fail at logical decision-making is that we mistake correlation for causation. If two variables are correlated, it doesn’t mean one caused the other. In fact, causation is less likely.
Here’s how mistaking correlation for causation results in misguided, irrational decisions.
Correlation Vs. Causation
Sometimes, we make unwise decisions because we assume that evidence shows causation when it really shows a correlation. When evidence reveals causation, it demonstrates that one variable causes another. When you base your decisions on causal evidence, you can feel confident that your decision will likely have a similar outcome.
By contrast, a correlation is evidence that two variables are related. However, just because two variables are related doesn’t mean that one causes the other. When you base your decisions on correlative data, you may make a wrong choice or waste your efforts.
For example, imagine you have a business that sells hand-knit sweaters. During your company’s first summer, you notice your sales are low. In response, you decide to invest extra time and money in marketing. When your sales surge from October to January, you attribute this increase to your recent marketing efforts. You then make this plan: Any time you notice a dip in sales, you’ll increase your advertising.
However, this plan may be a waste of your time and energy: It’s possible that your advertising efforts and your increase in sales may only be correlated. Perhaps your surge in sales from October to January was due to the fact that people typically buy more sweaters during colder months, not due to your advertising efforts.
How to Distinguish Causal Data from Correlative Data While Bevelin suggests that you should base your decisions on data that reveal causality, he doesn’t provide specific guidance on how to distinguish causal data from correlative data. In Cribsheet, economist Emily Oster provides additional guidance on how to improve your logical decision-making consulting research that reveals causation. (While Oster writes about improving parenting decisions specifically, her guidance is arguably applicable to any decision.)According to Oster, you should base your decisions on results from randomized trials instead of results from observational studies. Let’s contrast these two types of studies and further explore Oster’s claim. What’s a randomized trial? This is a type of experiment that reveals causation. In randomized trials, researchers randomly sort test subjects into groups, then they select one group to experience the variable under study. This process ensures that there are no other differences between the groups other than that variable. Therefore, these types of studies provide strong evidence that the variable studied causes the outcome that researchers observe. What’s an observational study? By contrast, an observational study tends to reveal correlations, not causation. This type of study compares groups of people without experimenting on them. Because these studies don’t involve an experiment, researchers can’t conclude whether the outcomes result from the participants’ actions or from other differences in their lives. These types of studies don’t provide strong evidence that the variable studied causes the outcome that researchers observed. How can you tell if a study is a trustworthy, randomized trial? According to experts, you should look for language (usually near the study’s introduction) that researchers randomly assigned participants to either an experimental group or a control group. |
A Solution: Base Your Decisions on Evidence That Shows Causation
To base your decisions on evidence that reveals causation instead of correlations, Bevelin recommends that you look for strong evidence that one variable causes another. For example, you could consult with other knitting entrepreneurs and compare data on when your sales rose and fell. Imagine that their sales also rose in the autumn, whether or not they ramped up their advertising efforts during that period. This comparison would reveal that there’s more evidence that the weather—not your marketing efforts—caused your autumn sales to increase.
Generate Your Own Causal Data Using a Trial Run Sometimes, it’s challenging to find strong evidence that one variable causes another. For instance, you may feel that you don’t have enough information to confidently conclude that the weather caused your sales to increase. Furthermore, it may be difficult to find research that relates to your situation. This lack of strong data may leave you feeling unsure of how to proceed with future decisions. In these cases, consider running your own experiment in the form of a trial run: a brief test in which you examine the effect of a single variable. As Dan and Chip Heath explain in Decisive, conducting a trial run of an option you’re considering for a decision provides evidence of an option’s outcomes. This can help you make an informed, rational prediction about how your decision may pan out long term. For example, one year you could run an advertising campaign in the fall, and the next year you could refrain from doing so. By comparing the two years’ sales, you’d gain some insight into whether the advertising campaign affected sales, and therefore whether you should invest in advertising during future fall seasons. |