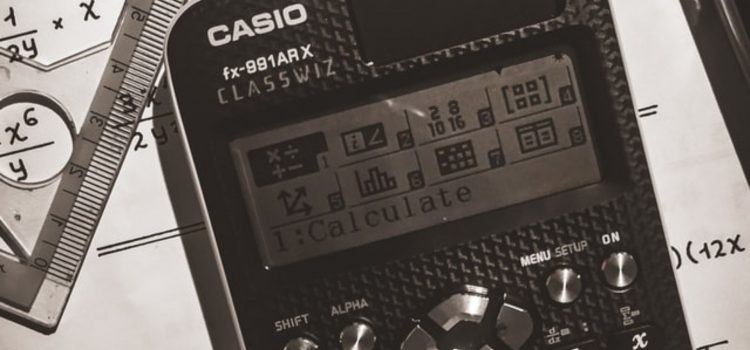
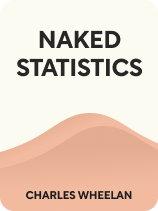
This article is an excerpt from the Shortform book guide to "Naked Statistics" by Charles Wheelan. Shortform has the world's best summaries and analyses of books you should be reading.
Like this article? Sign up for a free trial here .
What is descriptive analysis? How do descriptive statistics help us make sense of data? What is the main pitfall of descriptive statistics as a research tool?
Descriptive statistics take information in a data set and condense it into a meaningful figure like an average or percentile. Descriptive statistics help us summarize and describe data, characterize relationships, and make predictions. While descriptive statistics can help us make sense of data, they should be used with caution: Descriptive statistics tell us what happened, but they don’t necessarily tell us why.
Keep reading to learn about the limitations of descriptive statistics.
Understanding the Limitations of Descriptive Statistics
When using descriptive statistics to summarize data, we always make trade-offs between complexity and utility. Any time we take data from the real world and condense it into a single value, we gain insight into the data as a whole but lose nuance or the “story” behind that data.
Consider the following example to understand the limitations of descriptive statistics: Say your local elementary school implemented a new reading program that improved overall student reading skills by 15%. Hooray! However, further analysis might show that those gains were concentrated in students from high-income families, and the reading skills of low-income students stayed roughly the same. In light of this more complete picture, it seems clear that the program needs to be modified.
As our reading program example shows, the descriptive statistics that we choose when summarizing data have a determining impact on the story the data tells. Above, the first story is that the school implemented a successful reading program. The second story is that the school implemented a reading program that only benefitted wealthy students, exacerbating existing gaps in reading skills. Therefore, it’s important that we’re intentional and thoughtful about the descriptive statistics we choose to use.
Equity in Data As artificial intelligence advances and data collection and interpretation become more computerized, holding onto the real-world nuance of data is increasingly important. Modern technology allows algorithms to analyze data and draw conclusions as reliably as human experts. For example, in 2016, an algorithm outperformed physicians in diagnosing skin cancers by analyzing over 100,000 photographs of moles and melanomas. Diversity advocates stress the need to consider the story and nuance of the data used to build new tools and ensure that it represents all populations the algorithms are meant to serve. If the data we put into computer-based algorithms is biased, or misrepresents or under-represents certain populations, its results will be similarly flawed. Using biased statistics to make medicine or social sciences decisions can exacerbate existing inequities because they will best serve the populations for which we’ve collected the most data (which often ends up being white populations). For medical conditions like diabetes, which are already more prevalent in minority populations, algorithms based on white patients are especially problematic because they will improve medical care for white patients while doing little for the populations they could benefit most. |
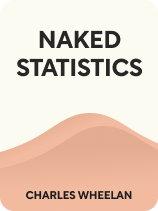
———End of Preview———
Like what you just read? Read the rest of the world's best book summary and analysis of Charles Wheelan's "Naked Statistics" at Shortform .
Here's what you'll find in our full Naked Statistics summary :
- An explanation and breakdown of statistics into digestible terms
- How statistics can inform collective decision-making
- Why learning statistics is an exercise in self-empowerment