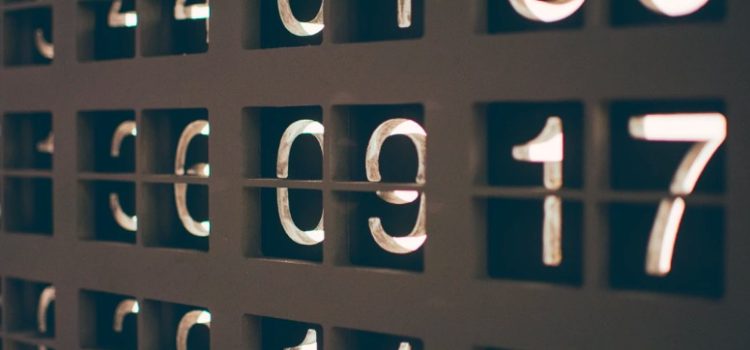
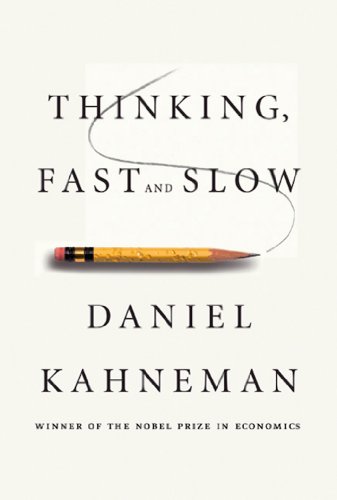
This article is an excerpt from the Shortform summary of "Thinking, Fast and Slow" by Daniel Kahneman. Shortform has the world's best summaries of books you should be reading.
Like this article? Sign up for a free trial here .
What is the law of small numbers? How does ignoring it lead to biased decision-making?
The law of small numbers is the bias of making generalizations from a small sample size. In truth, the smaller your sample size, the more likely you are to have extreme results. If you’re not aware of this principle, when you have small sample sizes, you may be misled by outliers.
We’ll cover two examples of the law of small numbers in action and how to use your awareness of it to make better decisions.
The Law of Small Numbers
A facetious example of the law of small numbers: in a series of 2 coin tosses, you are likely to get 100% heads. This doesn’t mean the coin is rigged.
In this case, the statistical mistake is clear. But in more complicated scenarios, outliers can be deceptive.
Law of Small Numbers and Decision-Making
Don’t be misled by small numbers.
The “Law of Small Numbers” Case 1: Cancer Rates in Rural Areas
A study found that certain rural counties in the South had the lowest rates of kidney cancer. What was special about these counties – something about the rigorous hard work of farming, or the free open air?
The same study then looked at the counties with the highest rates of kidney cancer. Guess what? They were also rural areas!
We can infer that the fresh air and additive-free food of a rural lifestyle explain low rates of kidney cancer; we can also infer that the poverty and high-fat diet of a rural lifestyle explain high rates of kidney cancer. But we can’t have it both ways. It doesn’t make sense to attribute both low and high cancer rates to a rural lifestyle.
If it’s not lifestyle, what’s the key factor here? Population size. The outliers in the high-cancer areas appeared merely because the populations were so small. By random chance, some rural counties would have a spike of cancer rates. Small numbers skew the results. This is the law of small numbers.
The “Law of Small Numbers” Case 2: Small Classrooms
The Gates Foundation studied educational outcomes in schools and found small schools were habitually at the top of the list. Inferring that something about small schools led to better outcomes, the foundation tried to apply small-school practices at large schools, including lowering the student-teacher ratio and decreasing class sizes.
These experiments failed to produce the dramatic gains they were hoping for.
Had they inverted the question – what are the characteristics of the worst schools? – they would have found these schools to be smaller than average as well.
When falling prey to the Law of Small Numbers, System 1 is finding spurious causal connections between events. It is too ready to jump to conclusions that make logical sense but are merely statistical flukes. With a surprising result, we immediately skip to understanding causality rather than questioning the result itself.
Even professional academics are bad at understanding this – they often trust the results of underpowered studies, especially when the conclusions fit their view of the world.
The name of this law comes from the facetious idea that “the law of large numbers applies to small numbers as well.”
The only way to get statistical robustness is to compute the sample size needed to convincingly demonstrate a difference of a certain magnitude. The smaller the difference, the larger the sample needed to get statistical significance on the difference.
Focusing on the Story Rather than the Reliability
Consider this result: “In a telephone poll of 300 seniors, 60% support the president.”
If you were asked to summarize this in a few words, you’d likely end with something like “old people like the president.”
You don’t react much differently if the sample were with 150 people or 3000 people. You are not adequately sensitive to sample size. You are misled by the law of small numbers.
Obviously, if the figures are way off (6 seniors were asked, or 600 million were asked), System 1 detects a surprise and kicks it to System 2 to reject. (But note weaknesses in small sample size can also be easily disguised, as in the common phrasing “6 out of 10 seniors”.)
Extending this further, you don’t always discriminate between “I heard from a smart friend” and “I read in the New York Times.” As long as you don’t immediately reject the story, you tend to accept it as 100% true. This is a variation of the law of small numbers.
———End of Preview———
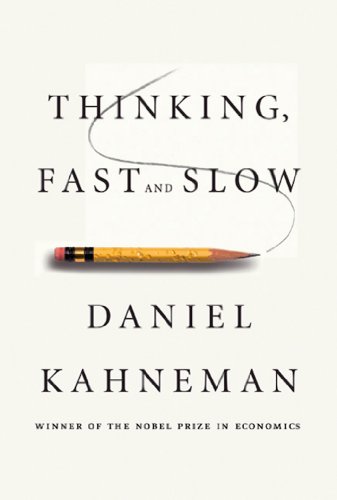
Like what you just read? Read the rest of the world's best summary of "Thinking, Fast and Slow" at Shortform . Learn the book's critical concepts in 20 minutes or less .
Here's what you'll find in our full Thinking, Fast and Slow summary :
- Why we get easily fooled when we're stressed and preoccupied
- Why we tend to overestimate the likelihood of good things happening (like the lottery)
- How to protect yourself from making bad decisions and from scam artists