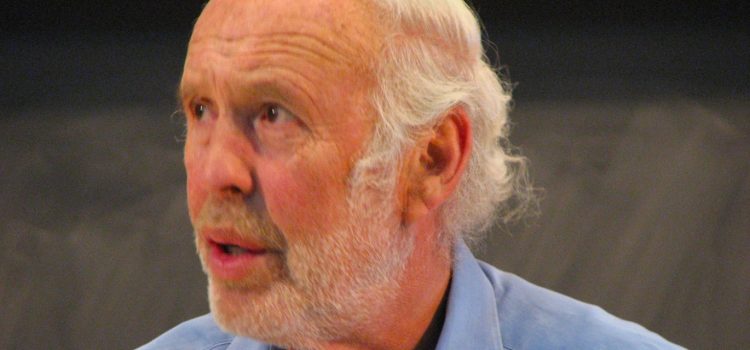
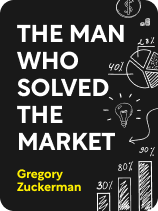
This article is an excerpt from the Shortform book guide to "The Man Who Solved the Market" by Gregory Zuckerman. Shortform has the world's best summaries and analyses of books you should be reading.
Like this article? Sign up for a free trial here.
What is Jim Simons’s trading strategy? Why did his strategy take off in the 1990s and 2000s?
Jim Simons’s trading strategy was so unique that it made him one of the best money managers in modern financial history. It focused on buying or selling currencies at the right time based on the model’s predictions of when they were most likely to rise or fall in value.
Let’s look at how the Renaissance strategy dominated the Quant Era of Wall Street.
Simons Hits His Stride and the Quant Era Begins
Jim Simons’s trading strategy proved prescient as computer power increased in the 1990s, writes Gregory Zuckerman in The Man Who Solved the Market. Since the computers could process more data, the fund’s models became stronger, faster, and more comprehensive. By the early 1990s, returns started hitting upwards of 70%—beating the market by a wide margin. By 1993, the fund was managing over $280 million. In this section, we’ll explore how Renaissance used machine-learning principles to refine its trading code and how Simons’s mathematics-based investing strategy eventually took over the finance industry.
(Shortform note: Renaissance’s exceptional growth during the 1990s may have been enhanced by the overall strength and productivity of the American economy during this time. Particularly as the decade drew into its second half, productivity growth surged to 2.5% per year—compared to 1.5% in the early 1990s. Economic historians attribute much of this productivity boom to investment in IT (across all sectors, not just in tech-intensive companies like Renaissance). Indeed, IT investment grew from 3% of GDP in 1991 to 4.9% by the decade’s end. As a consequence, innovation more than doubled in the second half of the 1990s.)
Mercer and Brown Join Renaissance
In 1993, writes Zuckerman, Simons hired computer scientists Peter Brown and Robert Mercer, renowned for their groundbreaking work in IBM’s language recognition unit. Simons recognized that these two scientists had experience and insight that could be of great value to Renaissance. This was because language recognition software depended on the same recognition of “states” in language as Renaissance’s model depended on recognizing market “states.” Just as a certain sequence of price movements could yield reasonable predictions about the next price movement, so could a certain sequence of words yield a reasonable prediction about the next word.
With their expertise in designing big systems, the pair were tasked with establishing a single trading code to handle Renaissance’s stock-trading business, constructing an optimal set of stock holdings given the fund’s risk appetite and financial resources. Best of all, Mercer and Brown designed the code based on machine learning principles: It would be adaptive, able to synthesize new information on the fly. The code was vastly more complex than the company’s previous code by several orders of magnitude, but it proved a winner for Renaissance: The fund was managing $900 million by 1997 and $5 billion by the mid-2000s.
The Growth—and Risks—of Automated Trading Algorithmic trading of the kind pioneered by Simons, Brown, and Mercer would sweep the financial world. Today, algorithmic trading accounts for around 60-73% of overall US equity trading. Despite its dominance, some financial experts observe that the spread of automated trading threatens to make the overall financial system riskier. Because financial markets (such as the stock market, the bond market, or the foreign exchange market) are often closely linked, algorithms that extend across markets can, in mere microseconds, inadvertently spread risk from one market to the next in a sort of “domino effect”—far faster than human traders could. Further, because of the speed at which these algorithms trade, a single flawed algorithm can quickly lead to catastrophic losses. In 2012, Knight Capital lost $440 million in 45 minutes due to a poorly written trading algorithm that executed millions of faulty trades in about 150 stocks. Ironically, despite claims about the “efficiency” of automated trading, Knight’s slip-up gave rival traders the opportunity to exploit the company’s tailspin as employees helplessly watched their algorithm spin out of control. |
The Quant Era on Wall Street
By the mid-2000s, the quant era had dawned on Wall Street. Rigorous mathematical models that analyzed the entire market were now the only way to beat the market. The financial world had at last taken notice of the emerging powerhouse fund run by the unusual collection of dressed-down mathematicians, computer scientists, and academics that was beating Wall Street at its own game. When Simons stepped down from Renaissance in 2009, he had amassed a personal fortune of $11 billion and rewritten the rules on Wall Street. His fund’s record of performance was untouchable, having consistently outperformed the market over nearly 30 years.
And in doing so, writes Zuckerman, Simons remade Wall Street in his image. The big firms that scoffed at his approach in the late 1970s and early 1980s had all to some degree or another adopted his quantitative and mathematics-based strategy by the dawn of the 2020s. From Fidelity to Merrill to the major banks, global financial institutions were investing the Renaissance way: absorbing megadata, building robust machine learning models to anticipate barely perceptible price movements, and creating computer models to automatically trade at scale based on those models.
Zuckerman writes that the datafication of everything will only make quantitative investing more powerful and dominant in the years and decades ahead. Data, after all, is the cornerstone of this investment strategy: And more data means more to analyze, more patterns to identify, and more tiny price fluctuations to capitalize on.
Does Data Collection Threaten Our Privacy? In The Age of Surveillance Capitalism, Shoshana Zuboff describes this drive for data collection by big business as a dark new frontier for the free enterprise system. She writes that this new form of capitalism—the titular surveillance capitalism—is defined by companies harvesting data about our behavior, making predictions about our future behavior using that data, and selling those predictions for profit. The idea is that serving people’s needs is less profitable, and therefore less desirable, than selling predictions about their future behavior. Zuboff warns that the ultimate goal of surveillance capitalism is to create a society in which our free will is replaced by behavioral conditioning that encourages predictable and machine-like patterns of behavior. At stake is our most basic right to privacy—our ability to exercise consent and control over our personal data and to not have the most intimate information about ourselves packaged and commodified. |
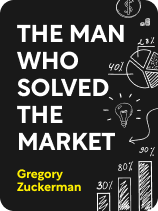
———End of Preview———
Like what you just read? Read the rest of the world's best book summary and analysis of Gregory Zuckerman's "The Man Who Solved the Market" at Shortform.
Here's what you'll find in our full The Man Who Solved the Market summary:
- The story of Jim Simons, one of the most successful hedge fund managers ever
- How Simons' experience as a codebreaker helped him succeed
- How Simons set the stage for today's large-scale, automated trading