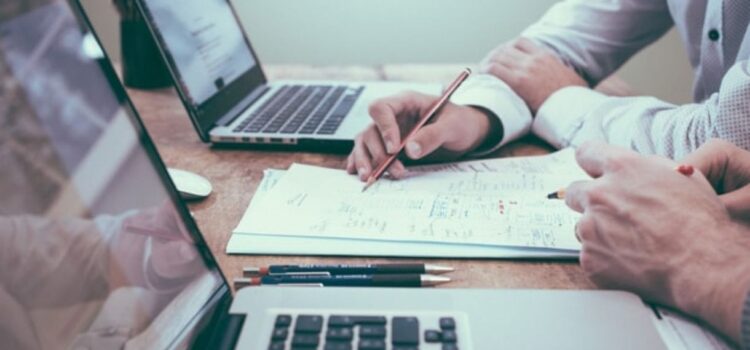
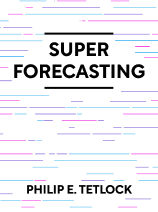
This article is an excerpt from the Shortform book guide to "Superforecasting" by Philip E. Tetlock. Shortform has the world's best summaries and analyses of books you should be reading.
Like this article? Sign up for a free trial here .
What is IARPA? When was it founded and what was its purpose?
The Intelligence Advanced Research Activity (IARPA) is an intelligence arm operating under the Office of the Director of National Intelligence. From 2011 to 2015, IARPA ran a forecasting tournament to identify forecasting professionals and gain insight into their methods.
Read about the IARPA forecasting tournament.
IARPA: Intelligence Advanced Research Activity
In 2006, the IC created a research arm called the Intelligence Advanced Research Projects Activity (IARPA). But IARPA quickly hit a snag: Useful research requires data, and they had no way to measure forecaster accuracy or track their methods.
To address this, IARPA officials approached Tetlock and his partner, Barbara Mellers, for help creating a forecasting tournament that would identify superforecasters and give researchers insight into their methods. Unlike the earlier EPJ tournament, forecasters would make predictions about events months into the future, not years, since forecasting accurately more than a year out is almost impossible.
The IARPA tournament was a bold move. If tournament results showed that a group of volunteers with no access to classified information made more accurate predictions than professional analysts, those analysts’ careers would be on the line. That type of bureaucratic boat-rocking is rare, even in fields with such high stakes.
Forecasting Tournament Results
The IARPA tournament was designed for researchers to glean insight into the statistical superforecasting methods. Armed with a much larger sample size, they could finally evaluate forecaster accuracy and compare forecasters to each other. Over the first two years of the tournament, Tetlock identified more “foxes” who scored better than 98% of the group. He dubbed them “superforecasters.”
In addition to identifying superforecasters, data from the multi-year IARPA tournament made it possible to track superforecasters’ performance over time and measure their regression to the mean. Surprisingly, superforecasters’ skills not only held up from one year to the next, they actually improved.
Remember, for a population this size, we expect to see regression to the mean, which would mean people in the top 2% would do slightly worse than the previous year, not better. The lack of any regression at all suggests that something is skewing the data. The authors think this happened because forecasters who did exceptionally well in year one were given the “super” designation and put on teams of other superforecasters for year two. It’s possible that this recognition provided a sense of accomplishment that inspired forecasters to work even harder—in the second year, superforecasters raised their individual scores enough to offset the usual regression. Over time, though, even skilled performers show some regression. After several years in the tournament, researchers determined the year-to-year correlation for individual scores is .65. In other words, the scores of about 30% of superforecasters regress toward the mean each year, while the other 70% remain “super.” If forecasting accuracy were purely a matter of luck, we’d expect 100% of superforecasters to regress to the mean over time. So we can confidently say skill plays a role for superforecasters—but what kind of skill?
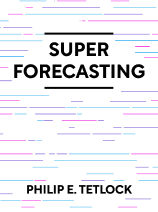
———End of Preview———
Like what you just read? Read the rest of the world's best book summary and analysis of Philip E. Tetlock's "Superforecasting" at Shortform .
Here's what you'll find in our full Superforecasting summary :
- How to make predictions with greater accuracy
- The 7 traits of superforecasters
- How Black Swan events can challenge even the best forecasters