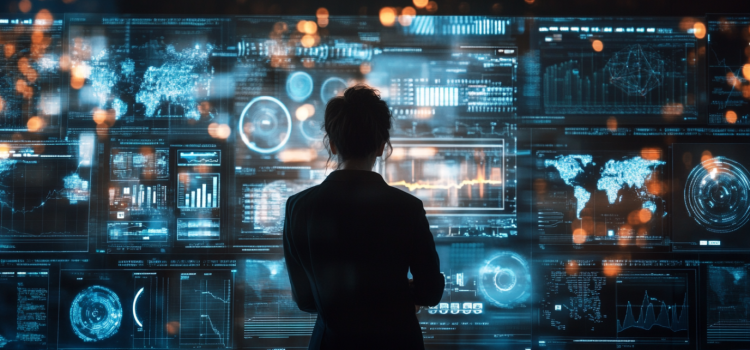
What’s the best way to make sense of those spreadsheets filled with thousands of numbers? How can you understand data in a way that helps you explain it to others?
In her book Storytelling With Data, Cole Nussbaumer Knaflic teaches readers how to interpret data by focusing on two essential skills. She offers practical techniques to transform complex information into clear, compelling visuals that anyone can understand.
Keep reading to discover how these strategies can help you become more confident in analyzing and presenting data of any kind.
How to Interpret Data
Imagine you’ve just received a massive spreadsheet containing your company’s global sales data. There are thousands of rows showing daily transactions across different regions, products, and customer segments. Your task is to present this information to your executive team next week. Where do you even begin? This is where data interpretation comes in. Knaflic says that, before you can create any visualizations, you need to make sense of what your data is telling you. You need to carefully examine your data to understand what’s significant and what’s just background noise.
Knaflic provides advice on how to interpret data effectively, explaining that it requires two key skills:
- Determining the appropriate level of detail for your audience—Do they need to see the forest or the trees?
- Understanding how to meaningfully compare different sets of data—Are you comparing apples to apples or apples to oranges?
Let’s look at each of these data interpretation skills in detail.
(Shortform note: Statisticians emphasize that, before you can present data, you have to understand the story behind it. Nathan Yau, author of Visualize This, explains that anyone can think through this task like a statistician by looking at the data objectively, without preconceived ideas, and asking why certain patterns, outliers, or inconsistencies appear. By questioning why the numbers look the way they do, you can uncover the explanations behind the data. Yau also explains that learning background information such as how the data was collected can help you interpret it more accurately and even uncover errors in the data, which are always possible where humans are involved.)
#1: Consider the Appropriate Level of Detail
First, consider the level of detail and specificity you need. Does your audience need to see big-picture trends or focus on specific numerical values? Knaflic says this will determine how you visualize your data: For example, you might label the axes of your chart to emphasize overall trends, trajectories, or patterns, but label granular data points to draw attention to specific values and help your audience see them in their context.
For example, imagine you work for a plant nursery, and you’re presenting sales figures for the different kinds of trees your company has sold over the past four quarters. To highlight the bigger sales trends and emphasize how different product lines—such as deciduous trees versus evergreen trees—compare with one another, you might make a simple line graph with axis labels. But if you need to scrutinize more granular sales numbers for a specific quarter, then you might make a bar graph that labels the specific data points individually, species by species.
In this example, the first graph provides a broad overview by summarizing total sales trends for deciduous and evergreen trees across four quarters. This high-level view makes it easy to compare the overall performance of these two categories and identify seasonal trends without getting lost in individual species data. Conversely, the second graph zooms in on the specific details by showing the exact sales numbers for each tree species in Q3. This level of detail is useful when analyzing individual species’ performance, making direct comparisons, or identifying which trees are driving category-wide trends.
(Shortform note: Other data scientists agree with Knaflic that interpreting data requires determining the appropriate level of detail. They point out that you have to choose which specific data points to visualize so you can highlight a particular narrative or insight. Lindsay Betzendahl (Visualizing Health and Health Care Data) explains that usually, you don’t want to represent all of the raw data in your presentation or visualization. Instead, you have to let go of unnecessary data points to focus on the most relevant information. That said, open-ended visualizations that include most or all of the data can also enable viewers to freely engage with the information and develop their own perspectives—after you’ve helped orient them.)
#2: Think About How to Compare Multiple Sets of Data
Second, when you’re working with multiple sets of data, you’ll face a classic visualization challenge: How do you show enough detail to be meaningful while avoiding information overload? Knaflic explains that our brains have limited visual processing capabilities, and too much information crammed together makes it difficult to focus on any single element. Therefore, you must find ways to present complex data that work with, rather than against, how our brains process information.
Knaflic offers three core principles for handling multiple data sets effectively. By applying these principles thoughtfully, you can create visualizations that reduce cognitive load and enable your audience to make sense of complex data without getting overwhelmed.
1. Use Consistent Scales
Knaflic says that, when comparing different data sets, using consistent scales makes it easy for your audience to interpret relative values and spot patterns. For example, if you’re tracking funding allocated to different nonprofit categories, using a consistent scale (such as 0 to 100%) across all categories allows viewers to quickly understand relative differences and trends.
2. Leverage Spatial Separation
Just as we naturally separate paragraphs in text to make reading easier, giving each data set its own space makes complex visualizations more readable. You can create this separation either vertically (using different rows or columns) or horizontally (using separate panels). This prevents the confusing effect of overlapping lines and helps your audience focus on one set of numbers at a time.
3. Know When Not to Compare
Sometimes, the most effective approach is to avoid comparisons altogether. Knaflic emphasizes that tracking trends within a single category often provides more meaningful insights than comparing across categories. For instance, if you’re analyzing social media engagement, looking at LinkedIn performance in isolation might reveal important patterns—such as posts performing better on Tuesday mornings—that would be obscured if you were comparing performance across all platforms.