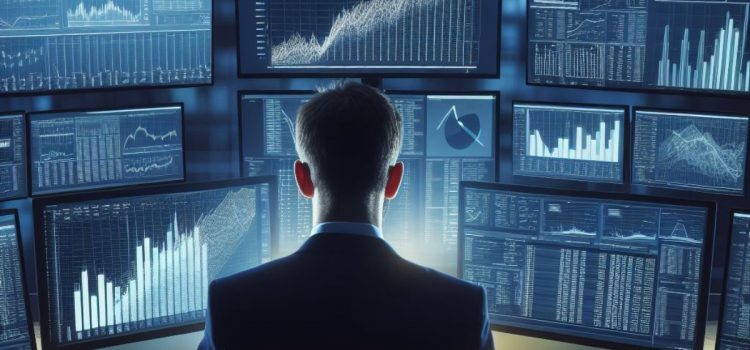
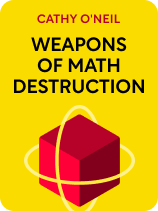
This article is an excerpt from the Shortform book guide to "Weapons of Math Destruction" by Cathy O'Neil. Shortform has the world's best summaries and analyses of books you should be reading.
Like this article? Sign up for a free trial here.
What’s the definition of mathematical models? Are math models biased toward a specific group of people?
In Weapons of Math Destruction, data scientist Cathy O’Neil details the insidious ways mathematical models are being used to determine everything from interest rates to prison sentences. She contends that, while mathematical models can efficiently sort through vast amounts of information, they can produce dangerous results.
Let’s take a look at O’Neil’s definition of a mathematical model.
Explaining Mathematical Models
According to O’Neil, this is the definition of mathematical models: mathematical simulations of a real-world event. Organizations use mathematical models to make predictions and decisions more efficiently than they would otherwise be able to when handling large amounts of data.
For example, suppose you recently adopted 100 cats, which you allow to freely roam your 100-acre property. To figure out where and when to leave food for the cats, you equip 20 of them with GPS collars to track their movements throughout the day. Based on the data from the collars, you estimate where the best places to leave food are—you’ve used data to create a simple mathematical model that simulates the movement of your cats without having to track all 100 cats.
(Shortform note: Mathematical models fall within the field of data science, which involves analyzing data, identifying patterns, and making predictions. Organizations increasingly rely on mathematical models with the explosive growth of big data—vast amounts of information that organizations collect from sources like social networks, electronic check-ins, and internet searches. Companies use this information to determine things like your spending habits and consumer preferences, which they then use in various ways such as targeting you with relevant ads.)
O’Neil argues that mathematical models are inherently reductive. Real-world events and people are immensely complex, and it’s impossible to input every factor that influences them into an equation. Lacking all the information necessary to simulate an event flawlessly, mathematical models inevitably produce a few mistakes. Organizations that use mathematical models are aware of these limitations, but they nonetheless use mathematical models because they’re willing to accept the trade-off between accuracy and efficiency.
To return to our example, your mathematical model of cat movements will occasionally return inaccurate results. As any cat owner can tell you, cats do what they want, when they want, coming and going in ways that aren’t entirely predictable. However, you decide that it’s still worth it to feed the cats based on the information your model spits out, as it’s much more efficient than tracking down and feeding each cat each day—and as long as all the cats are getting enough food, there’s no harm done.
(Shortform note: Though, as O’Neil argues, mathematical models are reductive, the trade-off is often worth it because models can solve highly complicated problems, offer many benefits in various industries, and even save lives. For example, mathematical models help keep miners safe by predicting roof collapses, help governments prepare for and respond to threats of infectious diseases such as Covid-19, and may be used to address climate change.)
O’Neil cautions that in addition to their limited accuracy, mathematical models also have ethical limitations. Mathematical models reflect the biases and incorrect assumptions of the people who make them. Additionally, unlike humans, mathematical models are unable to factor in moral and ethical concepts like equality.
For example, a cat feeding model designed by someone who incorrectly assumed that cats spend most of their lives in trees would probably place food sources up high. Based on this model, you’d end up distributing food unfairly, placing it where only the most nimble and adventurous cats would have access to it, while their terrestrial siblings would be left to fend for themselves.
(Shortform note: What kind of biases might someone unknowingly include in a mathematical model? In Decisive, Dan and Chip Heath cite four unconscious biases that compromise people’s decision-making: 1) binary thinking, which is our tendency to consider only two options, 2) confirmation bias, which is our tendency to search for information that supports our beliefs, 3) status quo bias, which makes us averse to change, and 4) hubris, which makes us overly confident in our predictions.)
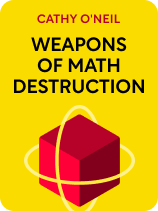
———End of Preview———
Like what you just read? Read the rest of the world's best book summary and analysis of Cathy O'Neil's "Weapons of Math Destruction" at Shortform.
Here's what you'll find in our full Weapons of Math Destruction summary:
- The insidious ways mathematical models are being used in society
- How mathematical models are being used to reinforce bias and harm the poor
- Recommendations for how to rein in dangerous mathematical models