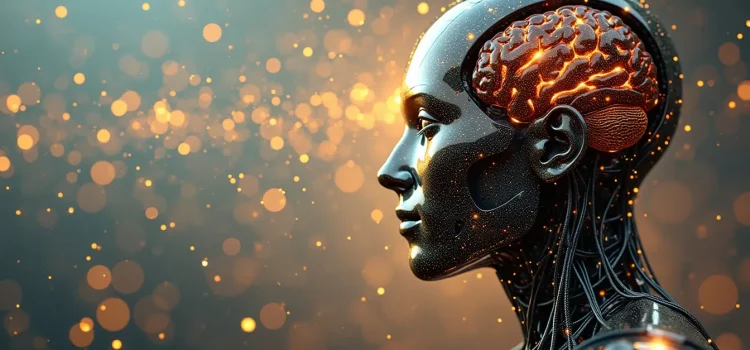
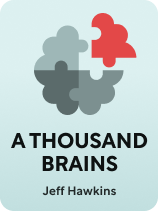
This article is an excerpt from the Shortform book guide to "A Thousand Brains" by Jeff Hawkins. Shortform has the world's best summaries and analyses of books you should be reading.
Like this article? Sign up for a free trial here.
What’s the future of artificial intelligence? How can our understanding of the human brain revolutionize AI?
According to Jeff Hawkins, AI development based on his Thousand Brains Theory could lead to exciting advancements. He proposes that AI systems should mimic the structure and function of the brain’s neocortex.
Read on to explore how Hawkins’s ideas might influence the future of AI.
From Neuroscience to AI
In addition to helping us better understand the human brain, the Thousand Brains Theory offers a promising avenue for advancing artificial intelligence research. According to Jeff Hawkins, AI researchers should shift their approach to more closely mimic the structure and function of the neocortex if we are to achieve Artificial General Intelligence (AGI)—a machine capable of learning and performing any intellectual task that a human can. There are still many advantages the human brain has over AI, but researchers could design new AI structures to mimic the model-building aspects of the neocortex, while discarding any aspects of the Old Brain that aren’t needed to build an intelligent machine.
While AI has proven successful in specific domains, such as pattern recognition and language generation, Hawkins points out that current AI development techniques are fundamentally different from how the brain operates. One key difference between brains and AI is that brains learn continuously, constantly updating their mental models based on new experiences. Modern AIs, on the other hand, are trained on fixed datasets before being put in use and don’t learn or adapt during operation. Additionally, human brains are capable of mastering a wide range of skills, while AI systems are typically designed for narrow, specific tasks.
(Shortform note: Hawkins isn’t the first to suggest that AI research can benefit by more closely modeling the processes of the brain. In Rebooting AI, Gary Marcus and Ernest Davis criticize current AI research for its narrow focus on “big data” and machine learning to the exclusion of other important tools, such as making use of current advances in neuroscience. While they don’t drill down to the level of cortical columns, as Hawkins does, Marcus and Davis assert that AGI will have to incorporate multiple processing systems and algorithms—just as the brain does—to interpret the real world’s multitude of inputs. AGI will also need a framework to mimic the brain’s understanding of how objects and ideas interact and change over time.)
To overcome AI’s current limitations, Hawkins suggests that researchers could develop AI architectures that create reference frames and mental models in a way similar to how the cortical columns of the neocortex process information. These AI systems would learn by actively exploring and interacting with their environment, building and refining models based on sensory input and motor feedback. Reference frames, which are already used in robotics, could enable AI systems to build models of knowledge and relationships rather than the current AI approach which relies solely on statistical probabilities.
(Shortform note: Inspired by Hawkins’s theory about the brain, researchers at Carnegie Mellon University are developing Cortical Columns Computing Systems that aim to replicate the neocortex’s structure in digital hardware. By integrating attributes of cortical columns and their reference frames into electronic neural networks, they hope to create energy-efficient processing units capable of continual learning. In addition to mimicking brain-like cognition, this approach seeks to achieve brain-like efficiency in computing, which could potentially lead to significant improvements in how much power and energy AIs use compared to current machine learning systems with large environmental footprints.)
To create truly intelligent machines, Hawkins says it won’t be necessary to replicate all aspects of the human brain. “Old Brain” functions, such as survival instincts and emotions, could be omitted or replaced with an “Old Brain equivalent” that handles low-level functions like movement and automatic reflexes. However, while AI doesn’t need animal traits such as hunger, fear, or the drive to reproduce, having a physical or virtual presence in the world is likely crucial for AI to develop true intelligence and motivations. By equipping AI with a variety of sensors and the ability to direct its attention as we do, researchers can give AI the necessary basis for learning and model-building in the real world.
Frameworks for Cognition In Rebooting AI, Marcus and Davis propose a software-based solution to replicate the natural instincts that Hawkins says AGI will need. Marcus and Davis suggest that AI researchers should focus on developing preprogrammed knowledge frameworks that could, for example, give AI a basis for connecting words to their meanings. A preprogrammed, instinctive grounding in real-world object relationships and context would let an AI learn on its own by expanding upon its preexisting knowledge. An AI would do this by exploring its environment in the context of its knowledge frames, much like the human brain does with reference frames. However, not everyone agrees with Hawkins that replicating human cognition is possible or even desirable. In Mind Over Machine and What Computers Still Can’t Do, philosopher Hubert Dreyfus argues that much of human intelligence and reasoning comes not just from the structure of the brain, but from cultural and experiential knowledge, which data-driven computers can’t emulate. Meanwhile, other researchers argue that we don’t need AGI at all because AI in its current form will soon surpass human abilities in many ways, and the belief that machines must think the way we do is a limited, human-centric attitude. |
The Dangers of Intelligence
The prospect of creating more powerful AI systems gives many people pause, but are the potential hazards of AI any worse than what humans are capable of on their own? Both AI and human intelligence pose potential risks to society, but the nature and scale of those risks differ. Hawkins argues that AI’s risks are often overstated, while human intelligence, with its primitive drives and capacity for self-deception, poses a more immediate and demonstrable threat to the world. In essence, we project our own human evils on machines that don’t have the basic primal urges that lie at the root of many human problems.
The concerns surrounding AI revolve around the possibility that it may become too powerful and autonomous for us to control. In one scenario, AIs create even more advanced AI that far surpass human comprehension, leaving mere human civilization in the dust. However, Hawkins doubts this is a valid concern—no matter how smart an AI becomes, the physical limitations of the real world would still constrain its ability to act and the pace at which it could acquire knowledge. After all, gaining knowledge requires conducting experiments and gathering data, both of which are limited to the pace at which physical research can be done.
(Shortform note: Despite how unlikely Hawkins says this scenario is, there are those who not only think it will happen, but who believe it will be a net-positive outcome. In The Singularity Is Near, futurist Ray Kurzweil argues that the rate of technological progress, especially in the realm of AI, will soon be accelerating so fast that it’s impossible to know what’s on the other side. Nevertheless, Kurzweil predicts there will be an intermediate “ramping-up” phase during which AIs will expand their knowledge base. If, during this time, we learn to use AI to augment human intelligence, then we ourselves will move forward as well, keeping pace with our computer counterparts as human intelligence shifts more and more into the digital realm.)
Another fear is that AI’s goals and interests may diverge from those of its human creators, leading to AI acting against human interests—the “robot apocalypse” frequently featured in dystopian science fiction. Again, Hawkins isn’t worried—this scenario rests on several unlikely assumptions, such as that AI systems would suddenly ignore the commands and inputs provided by humans, and that the AIs would have unchecked access to enough physical resources to carry out their sinister plans. Hawkins also notes that in humans, the human drives behind such behavior arise in the impulses of the Old Brain, while an AI based on the human neocortex wouldn’t share those motivations unless explicitly programmed to do so.
(Shortform note: Though Hawkins argues that AI won’t turn against humans the way other humans do, that doesn’t mean that AI shares humanity’s interests by default. Embedding human values into AI has become a significant challenge for companies developing AI-based products. First, they must explicitly define what those values are—for instance, human privacy and safety. Developers must design ways to limit AI behavior while establishing oversight mechanisms to ensure that AIs act as they should. Until AI is truly intelligent, we have to assume that it can fail in harmful ways, if not by design then by accident, just like any other tool.)
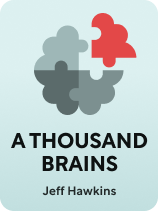
———End of Preview———
Like what you just read? Read the rest of the world's best book summary and analysis of Jeff Hawkins's "A Thousand Brains" at Shortform.
Here's what you'll find in our full A Thousand Brains summary:
- Why we need updated models for brain research
- How a new theory about human intelligence could be used to advance AI research
- Why human intelligence poses a more immediate threat to the world than AI does