What is the difference between mean and mode? Which of the two is a more accurate measure? Both the mean and the mode are measures of central tendency. For non-skewed distributions, the mean is more accurate because it takes into account every value in the data set. For skewed data, the median is better because it isn’t influenced by outliers. Keep reading to learn about the difference between mean vs. mode and when to use which.
Mean and Mode: Which Should You Use?
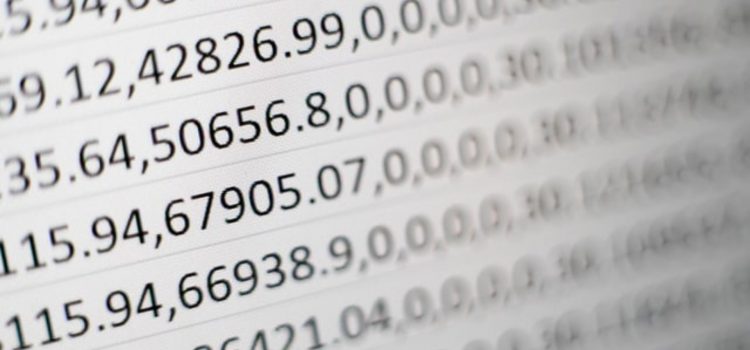