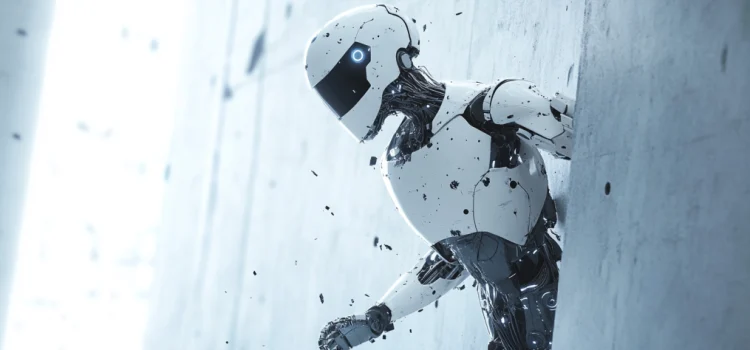
Are you worried about the future of AI? Have you ever wondered if we could simply stop its development? Can AI be controlled or contained?
In Scary Smart, author Mo Gawdat explores why halting AI’s progress is no longer possible. He explains that we’ve reached a point of no return, where superintelligent AI will become an independent, thinking entity beyond our control.
Keep reading to discover the three reasons why AI can’t be contained or controlled.
Controlling and Containing AI
If experts expect AI to create these dystopian scenarios or others, then why can’t we just put the brakes on further development? Can AI be controlled or contained? Gawdat explains that we’ve reached a point of no return, and we can’t stop these outcomes (and others like them) from occurring. He points out that superintelligent AI won’t just be a tool we’ve built: It will be an intelligent being that can learn, think, and decide just like we can. That means that we can’t control artificially intelligent systems in the same way that we can control more traditional computer programs—a scary thought if you’ve ever watched a film like 2001: A Space Odyssey.
(Shortform note: In predicting that specific outcomes of AI development are inevitable, Gawdat engages in what some call “technological determinism.” This involves arguing that if we build a technology like AI that’s smarter than humans, then the changes we envision it making to our culture are a foregone conclusion, good or bad. It’s equally deterministic to promise that social media will make the world “more open and connected” or to warn that AI will destroy humanity. Some observers say that while advances like AI make specific versions of the future more likely than others, they don’t, on their own, determine what the future will be.)
Gawdat explains that there are three fundamental reasons that we can’t put the genie back in the bottle (or the computer back in the box): It’s impossible for us to halt the development of AI, the code we write doesn’t determine how AI behaves, and we have no way of understanding how AI (even the models we have now) make their decisions. We’ll explore each of these ideas next.
It’s Too Late to Stop AI’s Progress
The first reason that AI can’t be controlled or contained is that we literally can’t stop its progress. Some people argue that we should stop developing AI for the good of humanity and the Earth. The goal would be to keep it from acquiring more robust thinking and problem-solving skills and progressing to artificial general intelligence.
But Gawdat contends it’s too late. Attempts to control AI development with legislation or to contain it with technological safeguards are up against the impossible because we’ve already imagined how we’ll benefit from more advanced AI. There’s immense competitive pressure among the corporations and governments pushing the development of AI forward and enormous economic incentives for them to continue.
(Shortform note: While there aren’t international laws restricting AI development, some experts say there should be. Many, like Gawdat, think it’s not possible to stop the progress, but that hasn’t stopped them from trying, as when 30,000 people signed a 2023 open letter calling for a moratorium on training powerful AI systems. Elon Musk, who signed the letter, has adopted an additional strategy for changing the direction that AI development is going. Early in 2024, Musk sued OpenAI, claiming it’s betrayed its original mission of developing open-source AI “for the benefit of humanity.” The lawsuit might be on shaky ground legally, but also practically: Some experts say the lawsuit is unlikely to affect AI development at OpenAI or anywhere else.)
Gawdat notes that some experts have suggested taking precautionary measures like isolating AI from the real world or equipping it with a kill switch we can flip if it behaves dangerously. But he contends that these proposals assume that we’ll have a lot more power over AI (and over ourselves) than we really will. Gawdat explains that we won’t always be smarter than AI, and we can’t depend on corporations and governments to curtail AI’s abilities at the expense of the potential gains of both money and power. He warns that we can’t stop artificial general intelligence from becoming a reality—and dramatically changing ours in the process.
(Shortform note: Gawdat isn’t the first to point out that we have a fraught relationship with our inventions, in part because we can’t keep ourselves from building things we shouldn’t. Mary Shelley’s Frankenstein, the 1818 novel that established science fiction as a genre, dramatized the idea that humans aren’t in control of the things we create. While Frankenstein is about the problem of playing God, AI has pushed writers to consider a new twist: the problem of creating God. Stories like HBO’s Westworld illustrate how our ability to develop technology outpaces our thinking on the implications of our inventions. As in Frankenstein, the artificially intelligent “hosts” in Westworld aren’t the monsters: The people who made them just to exploit them are.)
The Code We Write Is Only a Small Part of an AI System
The extent to which artificially intelligent systems depend (or, more accurately, don’t depend) on our instructions explains a second reason that much of AI’s behavior is out of our hands. Gawdat explains that for classical computers, how a machine operates and what it can do is explicitly determined by its code. The people building the system write instructions that tell the computer how to process the data it receives as input and how to complete the operations to generate its output. When systems operate in this deterministic way, they don’t need intelligence because they don’t make any decisions: Anything that looks like a decision when you use the program is determined by the instructions written into the code.
Gawdat explains that the unambiguous relationship between the code that controls a machine and the work that results from that code doesn’t apply to artificially intelligent machines. It all changed when researchers developed an AI method called deep learning, which enables AI to learn to complete a task without explicit instructions that tell them how to do it, learning in a way inspired by the human brain. (Shortform note: Deep learning is part of machine learning, a kind of AI that enables machines to learn from their experiences as humans do.)
As Gawdat points out, humans learn by taking in large amounts of information, trying to recognize patterns, and getting feedback to tell us whether we’ve come to the correct answer. Whether you’re a child learning to recognize colors or a medical student learning to distinguish a normal brain scan from a worrying one, you have to see a lot of examples, try to classify them, and ask someone else whether you’re right or wrong.
Deep learning enables AI to follow a similar learning process but at exponentially faster speeds. This has already made AI more skilled at detecting colors and identifying brain tumors than many humans. Instead of relying on explicit instructions that tell it how to categorize colors or how to spot a brain tumor, AI learns for itself by processing vast amounts of information and getting feedback on whether it’s completing a task satisfactorily.
What Is Deep Learning? Deep learning wouldn’t be possible without neural networks, the kind of AI that mimics some traits of the human brain. While some people use “deep learning” and “neural network” interchangeably, they don’t mean the same thing. A simple neural network needs just three layers of neurons or nodes: one to receive data, one to process it, and one to decide what to do with it. But a deep neural network has more than three layers, including “hidden” layers that transform the data. The process of training this sort of neural network is deep learning: The model trains itself on the data you give it. As Gawdat notes, this makes it possible for AI to teach itself to detect patterns instead of relying on explicitly coded rules. A deep neural network learns to see patterns using what researchers call a latent space. To tell apples and oranges apart, for instance, a model has to learn those fruits’ features and find a simple way to represent them so it can spot patterns. It does this in a kind of map, called a latent space. Similar images (like two images of apples) are closer together than very different images (one of an apple and one of an orange.) The idea of latent space was creepily mythologized when an artist said she “discovered” a woman she named “Loab,” haunting the hidden layers of an AI image generator. The artist asked for the opposite of Marlon Brando and ended up with uncanny images of a woman who’d look right at home in a horror movie. |
Gawdat explains that sometimes when a developer builds a program to complete a task, they don’t just build one AI model. Instead, they build thousands, give them large amounts of data, discard the models that don’t do well, and build updated models from there. Initially, the models complete the task correctly only about as often as random chance dictates. But successive generations of models get more and more accurate. The AI improves not because the underlying code changes but because the models learn and adapt. This is great for making AI that’s quick to learn new things. But it means that the initial code plays a smaller role than you might expect, and we don’t have control over how artificially intelligent machines learn.
What Is Evolutionary Learning? How Does It Compare to Deep Learning? In explaining how a developer might build thousands of models to end up with one, Gawdat describes “evolutionary learning” or “evolutionary computing.” This kind of AI differs from deep learning in a crucial way: Deep learning teaches a model something we already know, like training it to distinguish between cars and school buses by showing it images of both kinds of vehicles. Evolutionary learning trains a model to find answers that don’t yet exist, like asking it to find the most efficient route for a school bus to take through a busy neighborhood. While deep learning systems are made of neural networks and, in some ways, emulate how the brain works, evolutionary learning mimics the process that shaped the human brain: evolution. Just as randomness plays a role in evolution, the code you start with in evolutionary learning is random: hundreds or thousands of randomly generated pieces of code. Each gets tested, and the best pieces become part of the next version. The code evolves, changing and improving with each generation. As Gawdat notes, the AI’s learning gives you many generations of models—which, in our example, yield better and better bus routes over time—and you don’t have to start with the correct answer to build them. |
We Can’t Tell AI How to Make Decisions—or What Values to Adopt
A third reason that Gawdat characterizes AI as beyond our control emerges from our inability to control how AI makes its decisions. He explains that developers control how they build and train a model. But they don’t tell the model how to make decisions. They also can’t untangle the logic the model follows to make its decisions or learn from the vast amounts of data it’s trained on.
(Shortform note: While Gawdat contends that we often have no idea how an AI model has arrived at the answer it gives us, not all experts see this problem as intractable. Some researchers are working toward “interpretability” or “explainable AI.” As with the human brain, it’s not easy to look at individual neurons firing or even know which neurons to look at and explain how a specific decision gets made. But many of us would feel better about AI if it were less like HAL in 2001: A Space Odyssey and more like TARS in Interstellar: transparent about its logic, programmable to be 100% honest with us—unlike current AI that can “lie” about its decisions—and, preferably, disinclined to murder the humans it works with.)
Gawdat explains that AI is also quickly and constantly learning things that we’ve never taught it. The process of training AI models depends on a crucial resource: data. When an AI model learns from a dataset, that doesn’t just make it better at the tasks we give it. New skills also emerge in sometimes unpredictable ways. (Shortform note: Experts agree with Gawdat that large models learn unexpected new skills—but perhaps not as quickly as you might expect. Some say that the impression that new skills emerge out of the blue comes down to how we measure a model’s ability: What looks like a considerable jump when measured with one metric looks like gradual progress when measured with another.)
Gawdat explains that the enormous datasets we use to train AI also make the models better at understanding who we are, how we behave, and what we value. Just as he predicts AI will develop human qualities like consciousness and emotions, Gawdat also expects AI will develop a sense of ethics. AI is learning about us and what we value by observing what we write, what we tweet, what we “like,” and what we do in the real world. These observations will shape its values—including its sense of what’s morally right and wrong—and its values will shape its decision-making process.
Gawdat argues that, by showing AI that our highest values are narcissism, consumerism, conflict, and a disregard for others and for all the other beings on our planet, we’re teaching AI to value the wrong things. He explains that we can’t simply tell AI to adopt different, kinder ethics than those we demonstrate. We have to teach it not by what we say but by what we do. Whether we can succeed in doing that will determine whether AI helps us build a more prosperous future for everyone or contributes to a future where all but the few who are already at the top are worse off than we are now.
(Shortform note: The sense of ethics that Gawdat says AI will need might be difficult to teach it. Against Empathy author Paul Bloom explains that the combination of reason and emotion in human morality is hard for AI to grasp. Models like GPT string words together based on probability, not by understanding what the words mean. AI can “parrot” moral values reflected in its training data, but experts say it won’t be easy to teach AI to agree with our values,” a goal called “alignment.” Bloom contends the messiness of our moral values is part of the problem: We do bad things that we consider good—narcissistic, materialistic, and violent things, as Gawdat notes—and rationalize them in messy ways that make it difficult for AI to understand.)