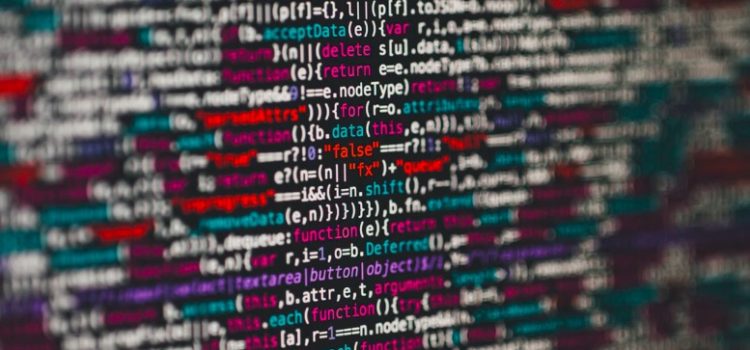
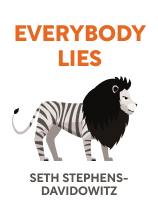
This article is an excerpt from the Shortform book guide to "Everybody Lies" by Seth Stephens-Davidowitz. Shortform has the world's best summaries and analyses of books you should be reading.
Like this article? Sign up for a free trial here .
What types of information does big data provide? How does it get this information?
In Everybody Lies, Seth Stephens-Davidowitz says big data opens our eyes to new types of information that weren’t previously available. We can find this new information from search engines and unconventional data sources.
Keep reading to learn where this big data information comes from.
Words, Words, Words
There’s nothing new about using words as data. As Stephens-Davidowitz points out, linguists, social scientists, historians, and others have studied words and word usage for a long time. But big data information dramatically enhances the type and volume of words researchers can study. (Shortform note: It’s this enhanced volume—another of the three Vs—that makes familiar data like text into big data. Thanks to the sheer volume of text databases and the ease of searching and comparing texts, it’s easier than ever before to study how words are used and combined across many different contexts.)
Stephens-Davidowitz himself bases most of his insights and arguments on search terms used in Google and other search engines. Search engines—and the databases of information they compile as users use them—are a relatively recent invention and represent a new source (or variety) of data for researchers and analysts.
Stephens-Davidowitz points out that computers also make it easy to analyze large volumes of text and/or speech that would be difficult or impossible to deal with manually. For example, he cites a study of word frequency in Facebook status updates and shows how word usage breaks down among gender and age lines—for example, perhaps unsurprisingly, he demonstrates that college-age people post about “studying” during the “semester” whereas 20-somethings drink “beer” when they aren’t “at_work.” Similarly, he explains that researchers can use sentiment analysis to determine the overall emotional tone of a body of text.
(Shortform note: While Stephens-Davidowitz is excited about how academic researchers can use text analysis—for example, he cites studies that use sentiment analysis to map narrative trajectories in works of fiction—most of the practical application of these techniques seems to take place in the business world. For example, businesses use text analysis and sentiment analysis to gauge customer interest and reactions, detect problems early, and improve customer service.)
Unconventional Data Sources
Though Stephens-Davidowitz specializes in using search records, text databases, and other similar types of data, he points out that there are new data sources that researchers have only recently started to tap. These data sources show that big data isn’t just about bigger databases of traditional information—it’s also about how new technologies let us turn more things into data by making them easy to measure, collect, compile, and analyze.
For example, Stephens-Davidowitz suggests that bodies can be data. He describes how consultant Jeff Seder helps buyers choose winning racehorses (Seder and his colleagues once advised a client to re-buy the horse he had just put up for auction—that horse was American Pharaoh, who went on to win the Triple Crown). Seder’s secret is that instead of looking at traditional horse judging factors like pedigree, he found anatomical factors (like left ventricle size) that correlate to race success.
(Shortform note: Body-based data is now far more prevalent than in Stephens-Davidowitz’s 2017 account. Whereas Seder’s work required him to custom build a portable ultrasound machine to examine horses, wearable fitness trackers and smart exercise equipment now allow many users to turn their bodies into data on a daily basis. Privacy experts have raised concerns over this trend, pointing out that these kinds of fitness products can record your biometric data, location, behavior patterns, and even your face and voice. In one notable case, maps based on Fitbit data revealed the location and layout of US military bases around the world and even showed the identities and workout routes of individual soldiers.)
Stephens-Davidowitz also argues that images can be data. For example, he describes how a company called Premise uses smartphone photos of things like gas station lines or grocery store produce to make economic predictions that are better and timelier than any traditional measures. What both images and biometric data have in common is that they’re easier to collect, compile, and study than ever before. Premise’s work is possible only thanks to smartphones, which guarantee that many people have high-quality cameras on their person at all times and which make it easy to share photographic data to a main hub from anywhere in the world.
(Shortform note: Whereas Premise contributors collect image data deliberately, other sources of image data are less obvious—and potentially more problematic. For example, Amazon’s smart doorbell Ring has come under fire for sharing video information with law enforcement without warrants. Similarly, a British judge recently ruled that a Ring doorbell violated a neighbor’s privacy due in part to its ability to record private conversations up to 68 feet away.)
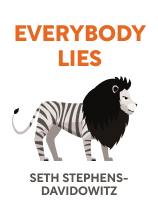
———End of Preview———
Like what you just read? Read the rest of the world's best book summary and analysis of Seth Stephens-Davidowitz's "Everybody Lies" at Shortform .
Here's what you'll find in our full Everybody Lies summary :
- How people confess their darkest secrets to Google search
- How this "big data" can be used in lieu of voluntary surveys
- The unethical uses and limitations of big data