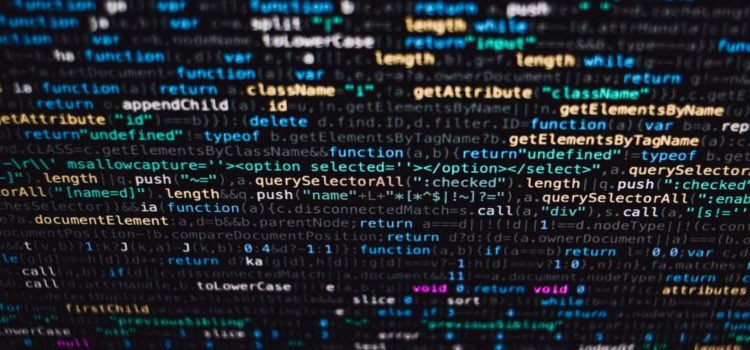
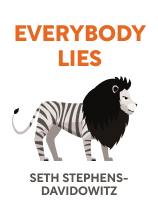
This article is an excerpt from the Shortform book guide to "Everybody Lies" by Seth Stephens-Davidowitz. Shortform has the world's best summaries and analyses of books you should be reading.
Like this article? Sign up for a free trial here .
What benefits does data science have that human intuition doesn’t? Why is data an extension of our intuition?
In Everybody Lies, Seth Stephens-Davidowitz looks at the bigger picture of what big data is and why we should care about it. Though data science might seem arcane, Stephens-Davidowitz argues that it’s an extension of our natural intuition.
Let’s look at the major benefits of data science that natural intuition doesn’t have.
Extending Our Intuition
Stephens-Davidowitz argues that when used well, data science is an extension of our natural intuition (though as we’ll see in a moment, it often defies our intuitive expectations and assumptions). He says that one of our basic activities as humans is spotting patterns and cause-effect relationships to make predictions.
Good data science, he says, is just an expanded, more rigorous version of this activity. However, there are two benefits of data science that natural intuition doesn’t have: It can consider much bigger sample sizes, and it doesn’t get distracted by compelling stories.
1. The Power of Bigger Samples
One of the problems with human intuition is that we can only base our judgments on our knowledge and experience. For example, a teacher grading an essay might suspect that a student copied material from a source. That suspicion often depends on previous knowledge—for example, how similar the paper is to previous plagiarism cases the teacher has encountered and how different the questionable passages are from that student’s previous writing.
Moreover, it’s hard to enforce plagiarism penalties based only on suspicion. But online plagiarism checkers—which compare the text in question to materials published on the web as well as to databases of other student papers—allow teachers to easily check questionable essays against millions of potential text matches.
(Shortform note: When it comes to human judgment, larger sample sizes (which often come with age and experience) can create overconfidence bias and prevent a person from recognizing deviations. For example, the longer a teacher teaches, the more likely she is to assume that she can recognize a cheater; this overconfidence can prevent the teacher from evaluating individual cases with thoughtful analysis.)
2. Defying Conventional Wisdom
According to Stephens-Davidowitz, another benefit of data science is that it isn’t beholden to conventional wisdom. Stephens-Davidowitz argues that humans are overly influenced by a good story, which leads us to make faulty predictions or explanations because they sound good or fit a preconceived notion. For example, sports commentators will often laud players for consistently getting clutch hits or hitting high-pressure shots—but data analysis suggests that clutch performance is primarily a statistical mirage rather than a repeatable skill.
(Shortform note: The narrative fallacy is our tendency to make predictions and conclusions based on stories rather than data. In Thinking, Fast and Slow, Daniel Kahneman explores the narrative fallacy and other related thinking errors at length. To overcome these inherent flaws in our thinking, Kahneman recommends learning to think statistically—and one way to do that is to augment our natural judgment with data science.)
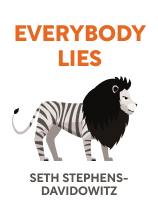
———End of Preview———
Like what you just read? Read the rest of the world's best book summary and analysis of Seth Stephens-Davidowitz's "Everybody Lies" at Shortform .
Here's what you'll find in our full Everybody Lies summary :
- How people confess their darkest secrets to Google search
- How this "big data" can be used in lieu of voluntary surveys
- The unethical uses and limitations of big data