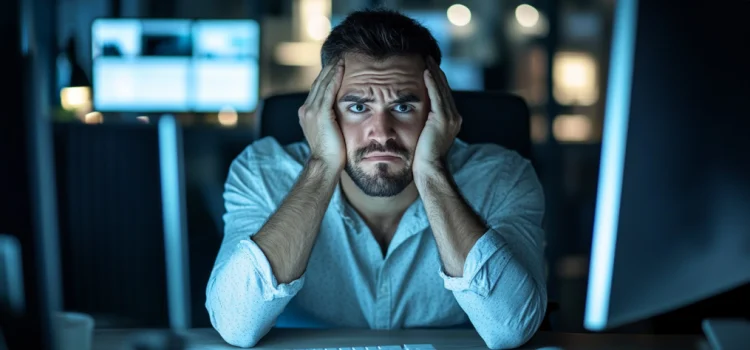
How capable is artificial intelligence in understanding human language? Why do AI assistants sometimes struggle with seemingly simple requests?
In their book Rebooting AI, Gary Marcus and Ernest Davis explore artificial intelligence and language. They argue that current AI systems, despite their impressive capabilities, lack a true understanding of human communication.
Read on to discover why your favorite AI assistant might not be as smart as you think and what it means for the future of human-machine interaction.
Artificial Intelligence and Language
What’s the problem with artificial intelligence and language? A great deal of AI research has focused on systems that can analyze and respond to human language. While the development of language interfaces has been a vast benefit to human society, Davis and Marcus insist that the current machine language learning models leave much to be desired. They highlight how language systems based entirely on statistical correlations can fail at even the simplest of tasks and why the ambiguity of natural speech is an insurmountable barrier for the current AI paradigm.
It’s easy to imagine that, when we talk to Siri or Alexa, or phrase a search engine request as an actual question, that the computer understands what we’re asking; but Marcus and Davis remind us that AIs have no idea that words stand for things in the real world. Instead, AIs merely compare what you say to a huge database of preexisting text to determine the most likely response. For simple questions, this tends to work—but if your phrasing of a request doesn’t match the AI’s database, the odds of it not responding correctly increase. For instance, if you ask Google “How long has Elon Musk been alive?” it will tell you the date of his birth but not his age unless that data is spelled out online in something close to the way you asked it.
(Shortform note: In the years since Rebooting AI’s publication, search engine developers have worked to include natural language processing into search algorithms so that search results don’t entirely depend on matching keywords in your queries. Natural language systems are trained to recognize the peculiarities of human speech, including common errors, “filler words,” intent, and context. The business sector is pushing research on stronger natural language systems thanks to the inherent flaws of keyword searching and the friction it causes for customers and stakeholders.)
A Deficiency of Meaning
Davis and Marcus say that, though progress has been made in teaching computers to differentiate parts of speech and basic sentence structure, AIs are unable to compute the meaning of a sentence from the meaning of its parts. As an example, ask a search engine to “find the nearest department store that isn’t Macy’s.” What you’re likely to get is a list of all the Macy’s department stores in your area, clearly showing that the search engine doesn’t understand how the word “isn’t” relates to the rest of the sentence.
(Shortform note: Guiding an online search using words like “isn’t” is a form of Boolean logic, a type of algebraic formulation that uses the conjunctions “and,” “or,” and “not” to determine whether a given point of data meets the requested search criteria. Boolean logic is a cornerstone of traditional computer programming which Davis and Marcus say has been discarded in the techniques used to train neural networks. Boolean terms can be used in search engines, but generally not via natural language. In Google, the words “and” and “or” must be typed in ALL CAPS to be used as operators, while the word “not” must be replaced by a minus sign to work, as in “find the nearest department store -Macy’s.”)
An even greater difficulty arises from the inherent ambiguity of natural language. Many words have multiple meanings, and sentences take many grammatical forms. However, Marcus and Davis illustrate that what’s most perplexing to AI are the unspoken assumptions behind every human question or statement. Given their limitations, no modern AI can read between the lines. Every human conversation rests on a shared understanding of the world that both parties take for granted, such as the patterns of everyday life or the basic laws of physics that constrain how we behave. Since AI language models are limited to words alone, they can’t understand the larger reality that words reflect.
(Shortform note: The underlying cause that Marcus and Davis hint at for AI’s lack of real-world understanding is that LLMs have no physical embodiment and interaction from which they can attach meaning and experience to the words and images they process. This isn’t true for other forms of AI, such as those that guide robots’ real-world interactions, but work to merge LLM technology with that of robotics is still in its infancy as of early 2024. If successful, these techniques may prove to be a big step toward teaching robots with AI how to infer meaning rather than relying on specific, spelled-out instructions for every minuscule action they take.)