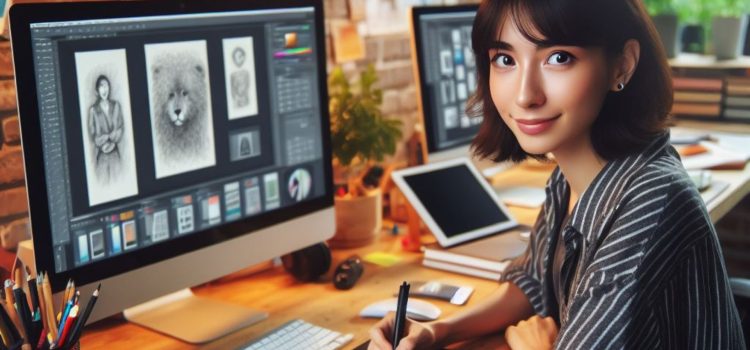
Are you concerned about the performance of AI systems in our daily lives? How can we ensure AI meets the same safety standards as other critical technologies?
In their book Rebooting AI, Gary Marcus and Ernest Davis explore the current state of AI standards and performance. They discuss the challenges of measuring AI capabilities and propose minimum requirements for AI reliability before we entrust it with crucial responsibilities.
Read on to discover why high AI standards are crucial for our future and what steps experts suggest we take to improve them.
The Importance of AI Standards
As AI permeates our lives more and more, the degree to which it functions well or poorly will have more of an impact on the world. However, because many AI applications have been in fields such as advertising and entertainment where the human consequences of error are slight, developers have grown lackadaisical about AI standards when it comes to performance. Davis and Marcus discuss their AI safety concerns, the difficulty of measuring an AI’s performance, and the minimum standards we should have regarding AI’s reliability before we hand over the reins of power.
In most industries, engineers design systems to withstand higher stressors than they’re likely to encounter in everyday use, with backup systems put in place should anything vital to health and safety fail. Marcus and Davis say that, compared to other industries, software development has a much lower bar for what counts as good performance. This already manifests as vulnerabilities in our global information infrastructure. Once we start to put other vital systems in the hands of unreliable narrow AI, a slipshod approach to safety and performance standards could very well have disastrous consequences, much more so than chatbot hallucinations.
(Shortform note: Some of the problems Marcus and Davis point out regarding the poor state of software engineering standards may be due to a mismatch between how corporate managers and computer programmers understand the process of software development. In The Phoenix Project, Gene Kim, Kevin Behr, and George Spafford demonstrate how efficient and productive software development gets hamstrung by a combination of unrealistic management expectations and engineers who lose sight of the business goals their work supports. Kim, Behr, and Spafford promote a production line model of IT work known as DevOps to bring the standards and practices of manufacturing into the world of software development.)
Exacerbating AI’s issues with performance, when AIs go wrong, they’re very hard to debug precisely because of how neural networks work. For this reason, Davis and Marcus are engaged in research on ways to measure AI’s progress and performance. One method they hope to adapt for AI is “program verification”—an approach that’s been used in classical software to confirm that a program’s outputs match standards and expectations. They also recommend that other AI designers explore a similar approach to improving performance, perhaps by using comparable AI systems to monitor each other’s functionality.
(Shortform note: Quantifying the performance of AI may actually turn out to be the easy part. We now have a variety of metrics to measure AI performance, accuracy, and precision, but determining whether AI is used ethically and safely is becoming a more prominent concern in the field. In 2023, US President Joe Biden signed an executive order directing AI developers and government agencies to share security data, establish safety standards, and protect the interests of consumers and workers through the economic changes brought about by AI.)
It would be unrealistic to expect any computer system to be perfect. However, the weakness of narrow AI is that, without human-level comprehension, it’s prone to unpredictable, nonsensical errors outside the bounds of merely human mistakes. Marcus and Davis insist that, until we develop stronger AI systems, people should be careful not to project human values and understanding on these purely automated systems. Most of all, if we’re to grant AI increasing levels of control, we should demand that AI have the same shared understanding of the world that we’d expect from our fellow human beings. We must avoid using a double standard.
(Shortform note: The discussion of how to give AI human values began in science fiction long before it became a practical necessity. Beyond the often-portrayed possibility of strong AI taking over the world, or at least displacing humans from whole sectors of employment, there are other ethical considerations, such as whose human values AI should be aligned with and whether self-aware AI should have legal rights. In The Singularity Is Near, Ray Kurzweil offers the view that, if strong AI is modeled on our human understanding of the world, it will include human values as part of its program, just as children learn values from their parents. It follows, then, that as we build strong AI, we must set the best example for it that we can.)