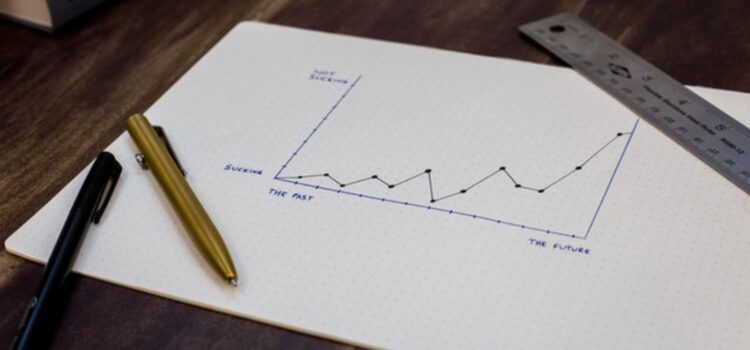
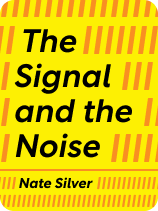
This article is an excerpt from the Shortform book guide to "The Signal and the Noise" by Nate Silver. Shortform has the world's best summaries and analyses of books you should be reading.
Like this article? Sign up for a free trial here.
Have you ever made a prediction that turned out to be wrong? What mental errors thwart accurate forecasting?
In The Signal and the Noise, Nate Silver examines mental errors that make your predictions inaccurate. These include making faulty assumptions, being overconfident, trusting data and technology too readily, seeing what you want to see, and following the wrong incentives.
Keep reading to learn how these mental errors cause inaccurate predictions.
1. We Make Faulty Assumptions
One of the mental errors that influence predictions is our tendency to make faulty assumptions. Our predictions tend to go awry when we don’t have enough information or a clear enough understanding of how to interpret our information. This problem gets even worse, Silver says, when we assume that we know more than we actually do. He argues that we seldom recognize when we’re dealing with the unknown because our brains tend to make inaccurate predictions based on analogies to what we do know.
(Shortform note: In Thinking, Fast and Slow, Daniel Kahneman explains that the brain uses these analogies to conserve energy by substituting an easier problem in place of a hard one. For example, determining whether a stock is a good investment is a complicated question that requires research, analysis, and reflection. Left to its own devices, your brain is likely to skip all that work and instead answer an easier question such as whether you have a good feeling about the company or whether the stock has done well recently. According to Kahneman, you can overcome this quick, associative reasoning by deliberately engaging your brain’s slower, more analytical faculties.)
To illustrate the danger of assumptions, Silver says that the 2008 financial crisis resulted in part from two flawed assumptions made by ratings agencies who gave their highest ratings to financial products called CDOs that depended on mortgages not defaulting.
- The first assumption was that these complicated new products were analogous to previous ones the agencies had rated. In fact, Silver suggests, the new products bore little resemblance to previous ones in terms of risk.
- The second assumption was that CDOs carried low risk because there was little chance of widespread mortgage defaults—even though these products were backed by poor-quality mortgages issued during a housing bubble.
Silver argues that these assumptions exacerbated a bad situation because they created the illusion that these products were safe investments when in fact, no one really understood their actual risk.
(Shortform note: These assumptions also highlight an especially pernicious thinking error called availability bias. In Thinking, Fast and Slow, Kahneman explains that availability bias occurs when we misjudge an event’s likelihood based on how readily we can think of examples of that event. This error is, in part, what led to the assumption that CDOs were safe. As Michael Lewis explains in The Big Short, investors and analysts repeatedly reasoned that because mortgages hadn’t tended to default recently, they were unlikely to do so going forward—even though the CDO bubble was created by an unprecedented laxity in lending practices.)
2. We’re Overconfident
According to Silver, the same faulty assumptions that make our predictions less accurate also make us too confident in how good these predictions are. One dangerous consequence of this overconfidence is that by overestimating our certainty, we underestimate our risk. Silver argues that we can easily make grievous mistakes when we think we know the odds but actually don’t. You probably wouldn’t bet anything on a hand of cards if you didn’t know the rules of the game—you’d realize that your complete lack of certainty would make any bet too risky. But if you misunderstood the rules of the game (say you mistakenly believed that three of a kind is the strongest hand in poker), you might make an extremely risky bet while thinking it’s safe.
(Shortform note: One way to avoid this overconfidence trap is to literally think of our decisions as bets. In Thinking in Bets, former professional poker player Annie Duke argues that most of the decisions we make carry some degree of uncertainty and therefore risk—after all, we rarely have complete information about any given situation. She argues that, as a result, making a good decision isn’t about picking the “right” choice or avoiding a “wrong” choice: It’s a matter of determining which option is most likely to give you the outcome you want. When you think this way, you can act knowing that you’ve given yourself the best chance of success—but that success isn’t a sure thing.)
Silver further argues that it’s easy to become overconfident if you assume a prediction is accurate just because it’s precise. With information more readily available than ever before and with computers to help us run detailed calculations and simulations, we can produce extremely detailed estimates that don’t necessarily bear any relation to reality, but whose numerical sophistication might fool forecasters and their audiences into thinking they’re valid. Silver argues that this happened in the 2008 financial crisis, when financial agencies presented calculations whose multiple decimal places obscured the fundamental unsoundness of their predictive methodologies.
(Shortform note: Whereas Silver argues specifically that precise predictions inspire overconfidence, in The Black Swan, Nassim Nicholas Taleb argues that numerical projections of any kind—even imprecise ones—tend to lead to (and reflect) overconfidence because random events make most predictions fundamentally unreliable given enough time. For example, Taleb cites forecasts from 1970 that predicted that oil prices would stay the same or decline in the next decade. In reality, events like the Yom Kippur War (1973) and the Iranian Revolution (1979) caused unprecedented oil price spikes during that period. Because it’s impossible to predict events like these, Taleb suggests that we should have very little confidence in predictions in general.)
3. We Trust Too Much in Data and Technology
As noted earlier, one of the challenges of making good predictions is a lack of information. By extension, the more information we have, the better our predictions should be, at least in theory. By that reasoning, today’s technology should be a boon to predictors—we have more data than ever before, and thanks to computers, we can process that data in ways that would have been impractical or impossible in the past. However, Silver argues that data and computers both present their own unique problems that can hinder predictions as much as they help them.
For one thing, Silver says, having more data doesn’t inherently improve our predictions. He argues that as the total amount of available data has increased, the amount of signal (the useful data) hasn’t—in other words, the proliferation of data means that there’s more noise (irrelevant or misleading data) to wade through to find the signal. At worst, this proportional increase in noise can lead to convincingly precise yet faulty predictions when forecasters inadvertently build theories that fit the noise rather than the signal.
Likewise, Silver warns that computers can lead us to baseless conclusions when we overestimate their capabilities or forget their limitations. Silver gives the example of the 1997 chess series between grandmaster Garry Kasparov and IBM’s supercomputer Deep Blue. Late in one of the matches, Deep Blue made a move that seemed to have little purpose, and afterward, Kasparov became convinced that the computer must have had far more processing and creative power than he’d thought—after all, he assumed, it must have had a good reason for the move. But in fact, Silver says, IBM eventually revealed that the move resulted from a bug: Deep Blue got stuck, so it picked a move at random.
According to Silver, Kasparov’s overestimation of Deep Blue was costly: He lost his composure and, as a result, the series. To avoid making similar errors, Silver says you should always keep in mind the respective strengths and weaknesses of humans and machines: Computers are good at brute-force calculations, which they perform consistently and accurately, and they excel at solving finite, well-defined, short- to mid-range problems (like a chess game). On the other hand, Silver says, computers aren’t flexible or creative, and they struggle with large, open-ended problems. Conversely, humans can do several things computers can’t: We can think abstractly, form high-level strategies, and see the bigger picture.
4. Our Predictions Reflect Our Biases
Another challenge of prediction is that many forecasters have a tendency to see what they want or expect to see in a given set of data—and these types of forecasters are often the most influential ones. Silver draws on research by Phillip E. Tetlock (author of Superforecasting) that identifies two opposing types of thinkers: hedgehogs and foxes.
- Hedgehogs see the world through an ideological filter (such as a strong political view), and as they gather information, they interpret it through this filter. They tend to make quick judgments, stick to their first take, and resist changing their minds.
- Foxes start not with a broad worldview, but with specific facts. They deliberately gather information from opposing disciplines and sources. They’re slow to commit to a position and quick to change their minds when the evidence undercuts their opinion.
Silver argues that although foxes make more accurate predictions than hedgehogs, hedgehog predictions get more attention because the hedgehog thinking style is much more media-friendly: Hedgehogs give good sound bites, they’re sure of themselves (which translates as confidence and charisma), and they draw support from partisan audiences who agree with their worldview.
5. Our Incentives Are Wrong
Furthermore, Silver points to the media’s preference for hedgehog-style predictions as an example of how our predictions can be warped by bad incentives. In this case, for forecasters interested in garnering attention and fame (which means more airtime and more money), bad practices pay off. That’s because all the things that make for good predictions—cautious precision, attentiveness to uncertainty, and a willingness to change your mind—are a lot less compelling on TV than qualities that lead to worse predictions—broad, bold claims, certainty, and stubbornness.
(Shortform note: In The Art of Thinking Clearly, Rolf Dobelli explains why we’re drawn to predictors who exude certainty and confidence. For one thing, he says, we dislike ambiguity and uncertainty—in fact, that’s why we try to predict the future in the first place. Meanwhile, we’re quick to assume that experts know what they’re doing (a phenomenon called “authority bias”) and we struggle to understand the probabilities involved in careful forecasts. Taken all together, these mental biases combine to make simplistic, confident predictions appear more convincing than more nuanced (but possibly more accurate) ones.)
Similarly, Silver explains that predictions can be compromised when forecasters are concerned about their reputations. For example, he says, economic forecasters from lesser-known firms are more likely to make bold, contrarian predictions in hopes of impressing others by getting a tough call right. Conversely, forecasters at more esteemed firms are more likely to make conservative predictions that stick closely to the consensus view because they don’t want to be embarrassed by getting a call wrong.
(Shortform note: Another reason forecasters might deliberately make bold predictions is to spur audiences into action. For example, in Apocalypse Never, Michael Shellenberger argues that some climate activists employ this tactic by deliberately making alarmist predictions in hopes of prompting policy and behavioral changes. The problem with this approach, Shellenberger says, is that it can backfire: In the case of climate change, if you successfully convince people that we’ve damaged the planet beyond repair, they might simply give up hope rather than enacting the change you’d hoped for.)
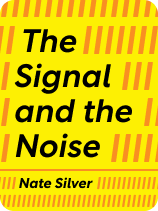
———End of Preview———
Like what you just read? Read the rest of the world's best book summary and analysis of Nate Silver's "The Signal and the Noise" at Shortform.
Here's what you'll find in our full The Signal and the Noise summary:
- Why humans are bad at making predictions
- How to overcome the mental mistakes that lead to incorrect assumptions
- How to use the Bayesian inference method to improve forecasts