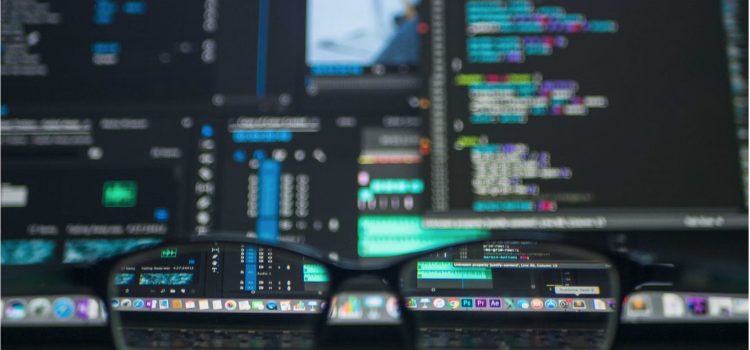
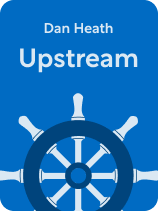
This article is an excerpt from the Shortform book guide to "Upstream" by Dan Heath. Shortform has the world's best summaries and analyses of books you should be reading.
Like this article? Sign up for a free trial here.
When you’re implementing change, what metrics should you monitor? How can you protect the process when data fails you?
In Upstream, Dan Heath explains how people can intentionally foster an upstream mindset to effect real change. He recommends several ways you can implement upstream solutions in any context. One of these is using data to guide experimentation.
Read more to learn about data-driven solutions and some challenges that come with the territory.
Data-Driven Solutions
One component of upstream intervention is using data to guide and tweak experimental solutions. The benefits of upstream problem-solving are not always immediately obvious. Heath writes that, in some areas (like preventive health care or changing a relationship dynamic), it could take years to see a significant change in outcomes. As a result, it’s important to be patient with the process and identify relevant metrics that you can continuously monitor—using data-driven solutions—to ensure that you’re headed in the right direction.
Heath contends that ample data allows you to not only identify early warning signs of a problem—to avoid being forced to react rather than prevent—but also allows you to track progress toward a goal and improve your strategy. For example, in the polluted stream scenario, relevant data might include the volume of trash on the banks of a particular stretch, the population of wildlife in the stream, or the number of families visiting the area (assuming that trash discourages both wildlife and humans from enjoying the stream).
An increase in the volume of non-biodegradable materials purchased locally might serve as an early warning sign that the problem will get worse, assuming that the prevalence of these materials contributes to the littering crisis. Heath suggests that trends in these areas could indicate success for the various stakeholders or indicate a need to change course. He emphasizes that you don’t have to predict the best solution from the beginning; you just have to respond accordingly to what the data shows.
Advice for Using Data Effectively In the context of personal development, research suggests that humans are generally bad at self-reporting progress toward a goal. This is because we tend to remember things as we expected or hoped them to be rather than making an objectively accurate assessment. In The Bullet Journal Method, Ryder Carroll recommends creating customized tracking sections in a monthly overview section of your journal to continuously monitor your progress toward goals. He contends that this strategy increases self-awareness and provides insight into which efforts are successful and which ones require modification. In The 12 Week Year, Brian P. Moran recommends tracking progress with two key indicators: lag and lead indicators. Lag indicators are the end results of your actions—the long-term outcomes you hope to achieve by taking upstream action. The lead indicators are the short-term actions taken to achieve that goal. For example, in the polluted stream scenario, the lag indicators would include the volume of trash in the stream and the lead indicators might include educational flyers posted about littering or the number of trash cans installed. Together, the two types of data help you understand both progress toward your larger goal as well as how effective your strategy is. |
Data-Related Challenges
Heath contends that there are multiple ways for data to fall short when it comes to accurately indicating the success of upstream efforts. Therefore, when implementing data-driven solutions, it’s important to constantly reevaluate the metrics you’re using to measure progress.
The first potential problem with data occurs when an overall trend driven by external factors gives the illusion that your specific efforts are driving change. For example, a manufacturing company might try to combat low customer satisfaction by beefing up quality control measures during the manufacturing process for products (an upstream solution). Customer satisfaction metrics might falsely indicate that these efforts are working even if the change was due to a personnel change in the customer support department.
Heath writes that the second data problem arises when the metrics for tracking upstream intervention don’t align with the overall goal. For example, in the customer satisfaction example, metrics related to quality control, like reducing the number of manufacturing errors, might not significantly contribute to customer satisfaction. A more accurate measure of success could be the number of negative reviews on the business’s retail website.
Lastly, Heath explains that a metric for short-term efforts can morph into the end goal rather than a means to a larger change. For example, if workers at a business get bonuses when there are fewer negative reviews for a product, reducing those numbers could become the top priority for the employees rather than improving the product and customer satisfaction. This could lead to people falsifying data or making it more difficult for customers to leave reviews, which is counterproductive to the long-term goal.
Heath suggests that one potential solution to this challenge is to pair two complementary metrics together to ensure that you’re moving toward the initial goal. For example, you might supplement the metric of negative product reviews with surveys on how individual consumers’ opinions changed over time.
A Business-Oriented Approach to Heath’s Data Problems The challenges that Heath discusses in this section are all related to the overarching problem of how to accurately measure and evaluate success toward a goal—a problem that business managers frequently wrestle with. In Built to Last, Jim Collins and Jerry I. Porras write that it’s important for a company’s policies and processes to reinforce the core values of the company to avoid any misalignment. They recommend staying vigilant and noticing when things like reward systems or organizational structure are slowing down progress toward the core goal. In Playing to Win, Alan G. Lafley and Roger Martin suggest a concrete strategy for businesses to successfully measure progress toward achieving a goal or solving a problem. They recommend giving each individual department within a company its own smaller goals to ensure that the strategy toward a larger goal is well-rounded. By using multiple metrics across different departments, the company can reduce the likelihood that one of the data problems Heath describes will derail the problem-solving effort. For example, if one department thinks it has achieved its goal, but it was actually a larger, external trend that caused a shift in its target metric, company-wide efforts toward the larger goal will continue across the other departments. Likewise, if one metric is misaligned with the larger goal or the metric itself becomes the priority, other departments’ metrics would compensate for it. Lafley and Martin’s recommendation for compartmentalized goals could also be adapted to Heath’s framework and applied in other contexts by giving each member or sub-group of the problem-solving team its own target metric for addressing root causes. |
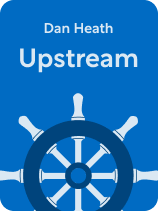
———End of Preview———
Like what you just read? Read the rest of the world's best book summary and analysis of Dan Heath's "Upstream" at Shortform.
Here's what you'll find in our full Upstream summary:
- Why you need to get to the root of a problem rather than applying band-aids
- Guidelines for implementing upstream solutions to solve problems
- How to solve problems before they happen